Beyond Self-Reports: How Clinical Psychologists Are Using AI for Deeper Behavioral Insights
Beyond Self-Reports: How Clinical Psychologists Are Using AI for Deeper Behavioral Insights - Expanding the view beyond questionnaires
While self-report questionnaires have served as a cornerstone in clinical psychology for decades, relying exclusively on them presents inherent limitations. They often struggle to fully encompass the fluid and complex nature of human behavior and internal states. To gain a more comprehensive understanding, the field is increasingly recognizing the necessity of looking beyond these traditional tools. This involves integrating different assessment strategies, moving towards collecting data from a wider range of sources. Exploring methods like passively monitoring behavior or incorporating observations from various perspectives offers the potential to gather richer, more objective information that self-reports might not capture or could even misrepresent. Such approaches aim to provide a more nuanced picture, potentially highlighting behavioral markers and emotional patterns that can inform clinical practice in ways previously difficult to achieve, though establishing the validity and practical utility of these alternative methods remains an ongoing effort.
Okay, looking into the potential beyond the standard questionnaire approach, particularly with computational tools, there are some interesting angles emerging. As a researcher curious about the practical application of these techniques in clinical settings, a few points stand out:
1. Utilizing data captured independently of direct self-report can sometimes highlight areas where a person's description of their experiences diverges from observable actions. Exploring these discrepancies, which might be difficult to surface through typical clinical conversation, could potentially offer insights into less conscious aspects of their presentation or coping patterns.
2. Computational methods are being explored to analyze simultaneous data streams – such as prosodic features in speech, subtle facial movements, or physiological indicators. The idea is to potentially pick up on fleeting or nuanced emotional signals during clinical interactions that human observation might not reliably catch, offering a potentially richer layer for understanding emotional processes, although validating these automated assessments is ongoing work.
3. Bringing in analysis of an individual's behavior and interactions as they occur outside the clinical setting, leveraging data from their everyday environment, could provide valuable context for insights gained during sessions or from questionnaires. This 'in-the-wild' perspective might help situate clinical findings within a more comprehensive picture of someone's life, which could inform more tailored support strategies.
4. The potential for automating certain data collection and initial analysis tasks, which are often time-consuming, is being investigated as a way to manage workload. If implemented effectively, this could potentially free up clinicians' time, allowing them to focus more on therapeutic engagement and complex decision-making rather than administrative data processing, assuming the tools integrate smoothly into workflows.
5. Analyzing behavioral patterns longitudinally, using algorithms to track changes over extended periods, shows promise for identifying subtle shifts that might indicate a person is moving towards a difficult period or potential relapse. While early detection would ideally enable proactive adjustments in care, establishing the reliability and clinical actionability of such indicators is still an active area of research, alongside navigating the associated privacy considerations.
Beyond Self-Reports: How Clinical Psychologists Are Using AI for Deeper Behavioral Insights - Turning diverse data into behavioral signals
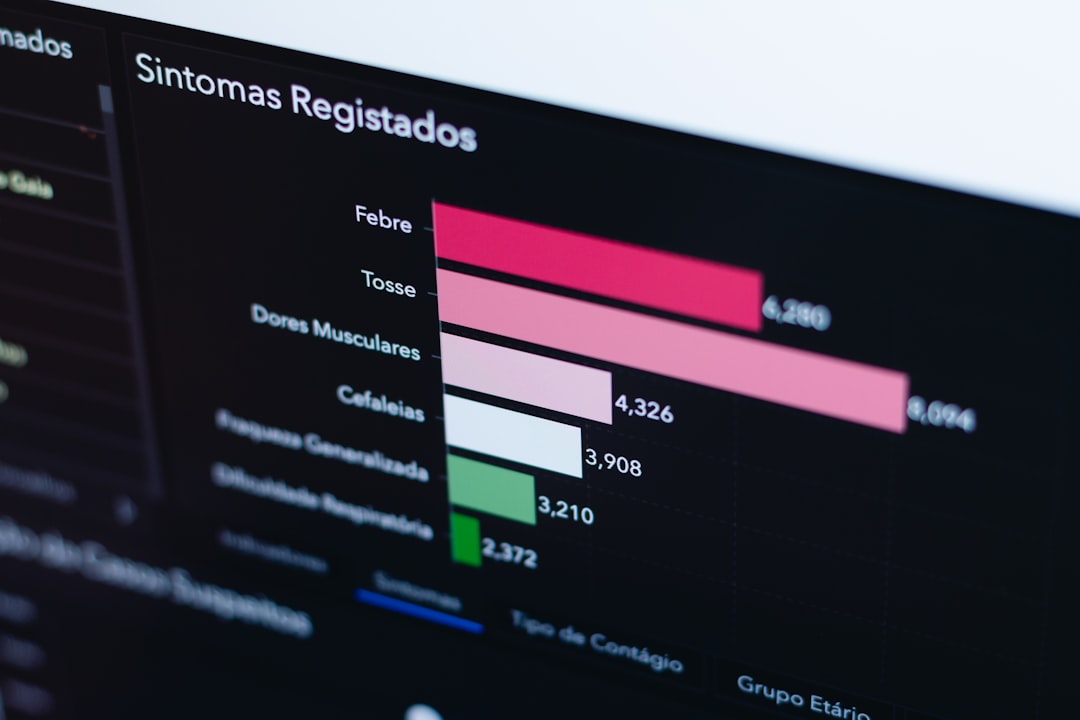
Extracting meaningful behavioral signals from a variety of incoming information marks a key evolution in clinical psychology. It allows for observing and understanding human actions and patterns in ways that go beyond simply asking people to describe their experiences. This process often involves the passive collection of data from ubiquitous sensors and digital tools. Applying machine learning techniques helps in learning from this data to identify subtle patterns – insights that might not be easily detected through direct observation or standard tools. However, significant practical challenges remain. These include ensuring the diverse data streams are compatible and can talk to each other (interoperability), navigating the often disparate metrics used, and establishing clear, reliable connections – correlations – between the measured digital signals and a person's actual mental health or behavior. Successfully addressing these technical and interpretive hurdles holds the promise of building a more comprehensive and contextually rich picture of someone's daily life and behavior. But translating raw data into clinically meaningful and actionable information requires rigorous validation and continuous refinement. Ultimately, while the capacity to derive these behavioral insights from varied digital information is evolving, ensuring data privacy, security, and overall integrity, alongside careful consideration of ethical use, is fundamental.
Okay, moving beyond the standard questionnaires and leveraging more diverse information streams, it's fascinating to consider what kinds of specific behavioral signals we might be able to extract and interpret using computational approaches. As someone interested in the nuts and bolts of turning raw data into meaningful insights, here are a few areas of potential exploration:
1. It's intriguing to explore how assembling information from varied sources—like interaction styles, activity levels, or physiological traces—might computationally distill into a unique behavioral signature for an individual. This goes beyond simply assigning a label, aiming instead to map out their specific ways of navigating the world, identifying potential behavioral assets or sticking points that might not fit neatly into established diagnostic buckets. The technical challenge involves harmonizing these disparate data streams effectively.
2. One line of inquiry explores whether patterns embedded in an individual's behavioral history and current data streams might hold clues about their likely response to specific therapeutic approaches. The hope is to eventually use these signals to inform more personalized intervention planning, ideally steering away from strategies less likely to be effective. However, building models that are both predictive *and* clinically trustworthy requires significant validation and understanding *why* a prediction is made.
3. From an analytical perspective, examining granular behavioral signals—like patterns in motor activity captured by passive sensors—raises the question of whether they might correlate with subtle shifts in cognitive processing. The idea isn't just identifying overt difficulties, but potentially noticing very early indicators of cognitive changes, perhaps even preceding what standard clinical assessments might catch. Establishing a robust link between these low-level physical or digital behaviors and cognitive function remains an active research area.
4. Exploring how to quantify aspects of social engagement through observable digital communication patterns presents an interesting technical challenge. By analyzing features like the frequency or diversity of digital interactions (appropriately anonymized and consented, of course), researchers are investigating whether a meaningful 'social connectedness' signal can be derived. This could potentially offer a complementary perspective to self-reported feelings of isolation or connection, providing additional data points for understanding a person's social world.
5. A technically interesting avenue involves designing interactive tasks, perhaps framed as games or simulations, where behavior can be meticulously recorded and analyzed by algorithms. The premise is that responses within these structured yet engaging environments might yield behavioral signals indicative of decision-making styles, risk tolerance, or emotional regulation in ways that traditional tests might not. Turning complex gameplay data into interpretable clinical insights is a key hurdle.
Beyond Self-Reports: How Clinical Psychologists Are Using AI for Deeper Behavioral Insights - Finding patterns the human eye might miss
The notion of finding patterns the human eye might miss is central to the growing use of computational methods in clinical psychology. This capability, enabled by AI, allows for the detection of subtle behavioral signals and relationships buried within complex data – information that is often too granular, too fast, or too interconnected for even a trained clinician to reliably perceive through direct observation or manual review alone. It signifies a new dimension in behavioral analysis, offering a different lens through which to explore the nuances of psychological experience.
Okay, picking up on how we might derive specific, subtle behavioral signals from diverse data streams, here are a few examples of the types of patterns researchers are exploring computationally, some of which might easily elude direct human observation:
1. Exploring the potential to detect extremely brief facial movements, sometimes called micro-expressions, which are typically too rapid for the human eye to consciously register. Algorithmic analysis of video data during interactions is being investigated as a way to computationally flag these fleeting signals, with the hypothesis that they might occasionally hint at emotional states or reactions that aren't being verbally expressed. Establishing the reliability and clinical significance of these subtle markers remains a technical challenge.
2. Investigating whether computational analysis of language patterns – looking at factors beyond just the words themselves, like sentence complexity, coherence over time, or changes in vocabulary richness in unstructured text or speech data – could potentially serve as an early indicator of very gradual shifts in cognitive function, perhaps even before changes are apparent in standard clinical assessments. Validating these subtle linguistic markers against clinical outcomes is a significant research task.
3. Examining whether passive data collected from wearable sensors related to sleep structure and patterns – things like how fragmented sleep is, or shifts in sleep timing and regularity – might offer clues about an individual's response trajectory to specific therapeutic interventions. The idea is to potentially use these objective, ongoing data streams to get a sense of whether a treatment appears to be having an impact earlier than relying solely on periodic clinical check-ins or self-reports, though translating these patterns into clinically actionable insights is not straightforward.
4. Analyzing acoustic features in speech, like variations in pitch contour, speaking rate, or the duration and placement of pauses during conversation. The hypothesis is that computational methods might detect subtle, possibly dynamic changes in these vocal characteristics that could correlate with shifts in emotional state or potentially indicate how an individual is responding to pharmacological treatment. The goal is to explore if these signals could provide finer-grained, potentially more frequent feedback loops for treatment adjustment, although disentangling clinical signal from normal variations is key.
5. Exploring whether subtle, non-verbal coordination or "synchrony" between individuals during clinical interactions – for example, mirroring of posture, tempo of movements, or turn-taking patterns in speech – might be detectable and quantifiable through computational analysis of audio or video. Research is investigating whether higher levels of this observed synchrony computationally correlate with stronger therapeutic relationships or are predictive of more favorable treatment outcomes, acknowledging that establishing causality and clinical utility is an active area of work.
Beyond Self-Reports: How Clinical Psychologists Are Using AI for Deeper Behavioral Insights - Implementing AI in everyday clinical work
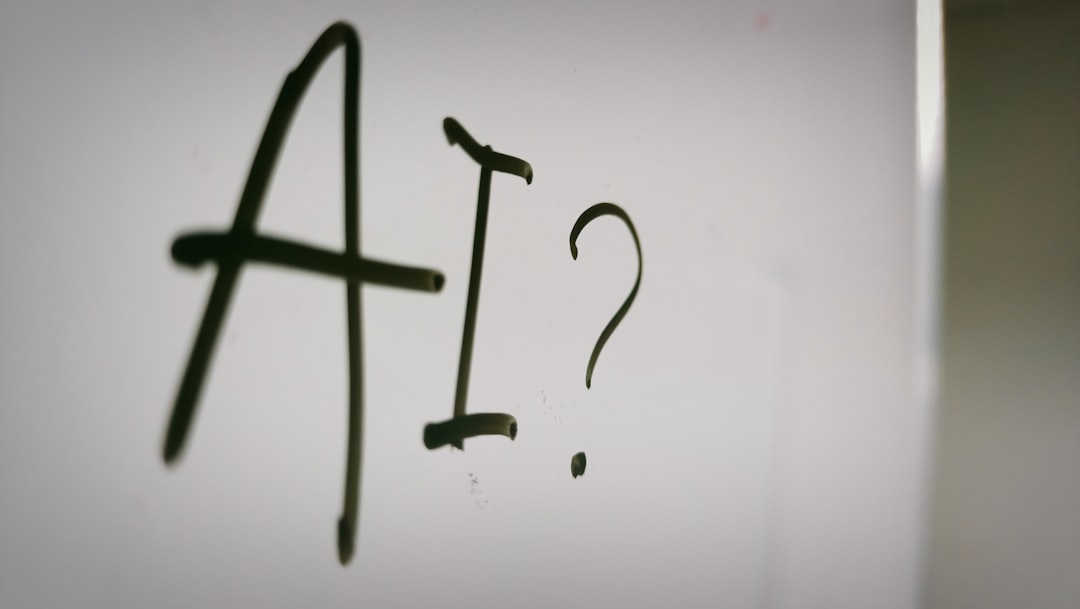
Weaving advanced computational capabilities into routine clinical psychology practice marks the current, evolving frontier. Beyond demonstrating potential, the immediate challenges involve navigating the practical complexities of integrating AI tools into existing workflows, ensuring clinicians can readily understand and utilize these insights, and building confidence in their reliability and ethical use within daily patient care. This ground-level operational shift, rather than just the analytical potential, is increasingly defining the landscape.
Okay, shifting the focus slightly to the practical challenges and possibilities inherent in bringing computational tools into the actual flow of clinical work, here are some areas researchers and engineers are exploring as of late May 2025:
1. Investigating the technical viability of integrating automated analysis of passively collected activity and sleep data – often from consumer wearables – into clinical review processes. The hypothesis is that identifying significant deviations or trends in these patterns might provide additional context or potentially flag periods requiring closer attention, though translating these data streams into clinically actionable insights within a busy practice workflow remains a key challenge.
2. Exploring whether computational linguistic analysis applied to routine clinical documentation or secure patient messaging could potentially reveal subtle, perhaps persistent, patterns in language that might correlate with aspects of a person's psychological state not always immediately apparent or easily self-reported during a session. The complexity lies in developing models robust enough to handle the variability of real-world clinical language and ensuring privacy during analysis.
3. Research is looking into the technical capacity of analyzing real-time digital interaction data during remote sessions – for instance, patterns in vocal pitch, speech rate, or even gross body movement captured by video – to computationally extract signals. The aim isn't to replace human clinical judgment but to investigate if these digitally derived features could potentially supplement a clinician's observations with quantitative data about interaction dynamics or physiological arousal.
4. Examining the feasibility of using consented, aggregated, and de-identified location and mobility data from smartphones to computationally map an individual's typical patterns of movement and social engagement over time. The technical hurdle involves identifying deviations from these baseline routines that might correlate with changes in well-being, with the intention of exploring their potential utility in informing discussions about behavioral activation or triggers, rather than attempting direct prediction.
5. Computational researchers are exploring if analyzing fine-grained features in an individual's voice captured during natural speech – such as changes in cadence, intonation variability, or articulation clarity over consecutive interactions – might statistically associate with subtle changes in neurological or cognitive function. Establishing the sensitivity and specificity of these acoustic markers for clinical purposes, particularly at very early stages, is a significant ongoing task.
Beyond Self-Reports: How Clinical Psychologists Are Using AI for Deeper Behavioral Insights - Considering the future of psychological insight
Considering the future of psychological insight means looking towards a landscape where our understanding is increasingly shaped by information captured beyond direct conversation or questionnaires. The integration of computational analysis, particularly artificial intelligence, opens doors to potentially perceiving subtle facets of behavior and internal states that have historically been difficult to access. This evolution represents a significant shift, one that offers the possibility of richer, more nuanced perspectives on mental health, but also requires careful consideration of the assumptions underlying these new methods and the responsible handling of the data they produce.
Okay, extending the discussion around integrating computational approaches and thinking about what might be revealed that's currently less visible, here are a few directions research and technical exploration are heading as of late May 2025, potentially uncovering insights that aren't immediately obvious through traditional methods or direct observation:
1. It's intriguing to explore how computational analysis might quantify subtle, non-verbal coordination between individuals during therapeutic interactions, perhaps within group settings or even between clinician and patient. Researchers are investigating if algorithms can pick up on patterns of synchronized movement, vocal rhythm, or even facial mimicry that might statistically correlate with aspects of relationship quality or group dynamics that are difficult for a human observer to consciously track consistently across multiple participants. The technical challenge lies in robustly capturing and interpreting these fleeting micro-behaviors across varied contexts.
2. Investigating the potential to leverage passive data streams from an individual's daily environment – like interactions with smart home devices or activity patterns inferred from consented sensor data – to explore correlations with behavioral indicators relevant to clinical goals, such as adherence to daily routines or potentially medication schedules. The hope is to computationally identify deviations from established patterns that might warrant clinical attention, though ensuring data privacy, security, and clinical interpretability of these environmental "footprints" remains a significant challenge.
3. Computational researchers are developing and testing methods that use controlled, interactive digital environments, like those in virtual reality, to probe reactions and behaviors under specific simulated conditions. The aim is to explore whether responses within these carefully constructed scenarios – such as decision-making styles or unconscious reactions to presented stimuli – might reveal less conscious behavioral patterns that are harder to access via self-report or observation in less controlled settings. Validating whether these simulated behaviors translate meaningfully to real-world clinical insights is a key hurdle.
4. Examining whether analyzing linguistic patterns and acoustic features in spoken language, perhaps captured during routine clinical interactions or via consented voice samples, could potentially reveal subtle, dynamic shifts in cognitive processing or emotional state over time. The focus is on picking up on fine-grained changes in fluency, complexity, intonation, or other vocal characteristics that might precede more overt clinical changes, although disentangling clinically relevant signals from natural variability and background noise is a substantial technical task. This also includes exploring if such analysis can account for linguistic and cultural diversity, which introduces further layers of complexity.
5. A line of technical inquiry explores using computational methods to analyze large volumes of historical behavioral data, potentially including sequences of past interactions or responses to interventions (with appropriate consent and de-identification), to identify complex patterns. The hope is to potentially build models that could offer clinicians insights into possible trajectories or statistically-informed perspectives based on historical data, helping to contextualize an individual's current situation, rather than aiming for direct prediction which carries significant ethical and safety concerns. The critical work here is ensuring transparency and trustworthiness of any computationally derived insights for clinical use.
More Posts from psychprofile.io: