AI-Powered Psychological Profiling - Gain Deep Insights into Personalities and Behaviors. (Get started for free)
Calculating Mental Health Prevalence A 7-Step Guide for Accurate Assessment
Calculating Mental Health Prevalence A 7-Step Guide for Accurate Assessment - Defining the Target Population and Scope
When estimating the prevalence of mental health conditions, defining the target population and study scope is absolutely crucial. The accuracy and usefulness of the research hinge on this foundation. A well-defined target population ensures that the individuals selected for the study genuinely represent the wider population grappling with mental health challenges. This is fundamental for developing effective policies and informing clinical decisions. With the increasing number of people seeking mental health support and the growing prevalence of disorders like anxiety and mood disorders, carefully defining target groups is crucial to understanding and addressing the existing gaps in care.
Adding to the difficulty, there's a noticeable lack of research proving the effectiveness of population-based approaches to mental health. This highlights the need for thorough and insightful data collection to guide healthcare strategies. By establishing clear boundaries for the target population, researchers can design more effective interventions and distribute resources strategically, which helps ensure these interventions reach the most vulnerable.
When we aim to understand the prevalence of mental health conditions, precisely defining who we're studying – the target population – is absolutely critical. The accuracy of our estimates hinges on this definition. A poorly conceived scope can drastically skew results, leading to an underestimation or overestimation of mental health issues within specific subgroups. For instance, if a study overlooks certain ethnicities or age groups, it won't offer a complete picture of the prevalence landscape.
It's fascinating how the inclusion criteria for studies on mental health vary so widely. What constitutes a 'target population' seems to be a moving target, depending on the specific research goals, the characteristics of the population being analyzed, and even the nature of the mental health condition itself. This variability makes comparisons across studies quite tricky.
Research consistently shows that mental health isn't uniformly distributed across different ethnic or racial groups. Prevalence rates tend to vary, indicating that a one-size-fits-all approach to mental health assessment might miss important nuances in the needs of diverse populations. It's imperative that researchers acknowledge these disparities when designing their studies.
The life stage of an individual significantly influences their vulnerability to specific mental health conditions. Anxiety may be more prevalent among young adults navigating life transitions, whereas depression might be more common in older adults facing unique challenges. It's important to be mindful of these age-related patterns when defining target populations and interpreting findings.
Economic status, too, is inextricably linked to mental health. Individuals facing economic hardship often report higher rates of mental health issues. This disparity highlights the need for tailored assessment approaches that consider the influence of socioeconomic factors.
Location – urban versus rural – can also impact mental health. Studies suggest that individuals living in urban environments may experience higher levels of stress and anxiety compared to those living in rural areas. It's worth investigating the complex interplay between geographical location and mental health when determining the scope of a study.
Gender differences in mental health prevalence are well-documented. Men and women tend to experience different types of disorders at varying rates. Understanding these disparities is crucial for developing targeted assessment and intervention strategies.
Longitudinal studies, which track changes in mental health over time, are invaluable. They enable us to observe how life events and broader societal shifts influence mental health prevalence. This type of research offers a richer understanding of the dynamics of mental health than cross-sectional studies.
Mental health stigma can be profoundly influenced by culture and can vary across populations. This variation impacts how individuals seek help and report their mental health conditions. This cultural context must be carefully considered when defining a target population.
Lastly, the rise of digital technologies in mental health assessment is revolutionizing how we understand and reach specific populations. Online surveys and other digital tools allow researchers to access a wider range of individuals than traditional methods. This expanding access opens new avenues for understanding mental health prevalence, but also requires thoughtful consideration of potential biases introduced by this technological shift.
Calculating Mental Health Prevalence A 7-Step Guide for Accurate Assessment - Selecting Appropriate Assessment Tools
Choosing the right assessment tools is crucial for accurately evaluating mental health, as these instruments must be tailored to the specific characteristics of the populations being studied. The selection process requires careful consideration of the tools' psychometric properties, which include their validity, reliability, and accessibility. It's essential that the chosen tools are backed by evidence and are appropriate for the particular setting, whether it's a clinical environment or a larger public health study. Because the nature and prevalence of mental health conditions can differ significantly among diverse demographic groups, it's vital to adjust assessment methods to accurately reflect the unique needs of each group. The rising prevalence of mental health disorders emphasizes the need for precise diagnostic tools to improve the accuracy of diagnoses and guide the development of effective treatment approaches. Therefore, making thoughtful and informed choices during tool selection is vital for promoting optimal mental health outcomes.
The selection of assessment tools plays a pivotal role in determining the prevalence rates reported in mental health research. If inappropriate tools are chosen, measurement bias can creep in, potentially leading to either an underestimation or overestimation of mental health conditions. This emphasizes the importance of ensuring that the tools are suitable for the specific population and research question.
It's crucial to verify the reliability and cultural relevance of any assessment tool. Tools created in one cultural environment may not translate effectively to others, possibly leading to misinterpretations of mental health prevalence across diverse populations. This highlights the need to consider how cultural norms and perspectives might influence responses to assessment tools.
A significant portion of mental health assessment relies on self-reporting, which raises concerns about potential bias from social desirability. Individuals might downplay their symptoms due to stigma or fear of negative consequences. This can paint a distorted picture of true mental health prevalence, underestimating the actual numbers.
The sensitivity and specificity of assessment tools can vary significantly. Using instruments with low sensitivity might result in overlooking a considerable number of cases, while tools with low specificity might lead to an excess of false positives. This can significantly complicate the interpretation of prevalence data and the discussion around it.
Initial screening tools are often employed to identify individuals who need further evaluation. However, these initial screens can generate a substantial number of false positives. This necessitates the utilization of more comprehensive and rigorous assessment methods later to arrive at accurate prevalence calculations.
Digital tools for mental health assessment, though revolutionary, have the potential to inadvertently exclude certain populations from study participation. Limited digital literacy or restricted access to technology in specific demographic groups can introduce bias into prevalence estimates. This underscores the importance of ensuring equitable access to digital assessment technologies.
The timing of assessments can also influence study findings. Mental health conditions can fluctuate over time, and assessments conducted at different periods might produce drastically different prevalence rates. This highlights the need for longitudinal studies that track changes in mental health over time for a more complete picture.
Traditional assessment tools often disregard social and environmental factors that can strongly influence mental health. This oversight can result in an incomplete understanding of how external influences impact mental health prevalence. Incorporating these variables into the assessment process is necessary for a more comprehensive view of the issue.
The implementation of assessment tools can be financially and time-consuming. This creates disparities in settings with limited resources, where access to high-quality assessments is vital for generating accurate prevalence data. Resource allocation and tool selection should consider the feasibility and affordability of implementation.
Finally, the format of the assessment – whether written, verbal, or digital – can influence both participation rates and the veracity of responses. This subsequently impacts reported mental health conditions and overall prevalence rates. Carefully considering the format of assessment can help reduce bias and improve data quality in prevalence studies.
Calculating Mental Health Prevalence A 7-Step Guide for Accurate Assessment - Gathering Representative Data Samples
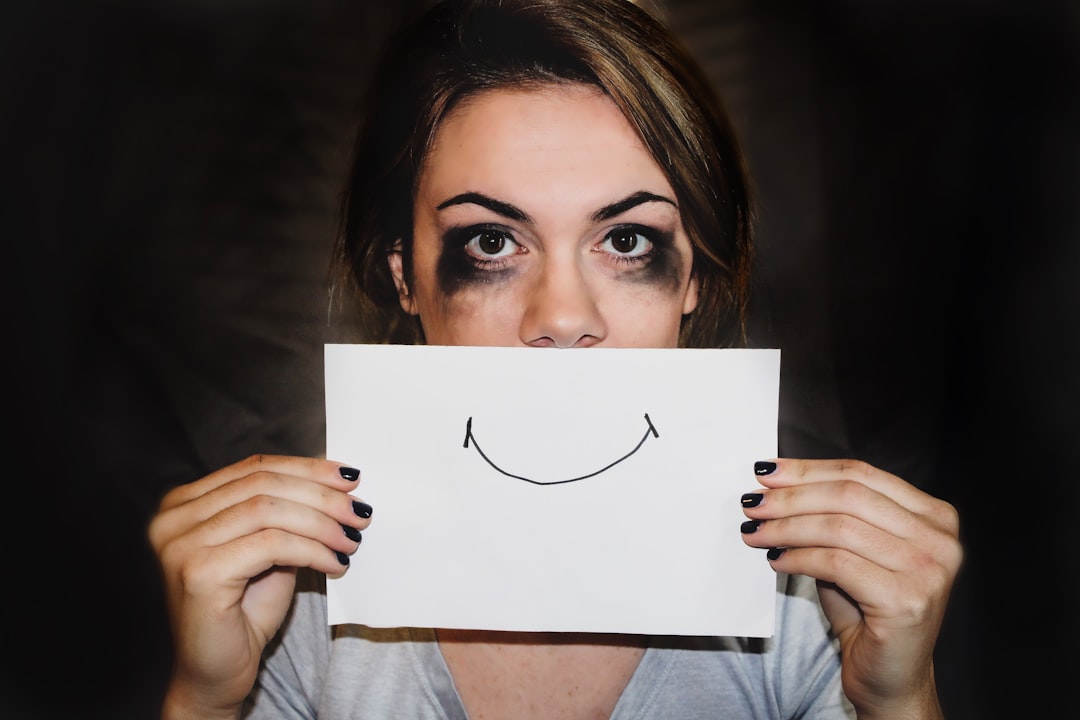
To accurately estimate the prevalence of mental health conditions, gathering representative data samples is critical. A representative sample reflects the characteristics of the larger population it aims to represent, allowing researchers to draw conclusions that are applicable to that broader group. This is achieved through careful use of probability sampling techniques, like random sampling, which helps ensure that diverse aspects like geographical location, socioeconomic status, and cultural backgrounds are proportionally represented within the sample. However, achieving representativeness can be a significant hurdle, as mental health conditions are not uniformly distributed across different populations. If these variations aren't considered, the results might not be accurate, potentially leading to misleading conclusions. The validity of mental health prevalence studies hinges on the quality of the data collection process, ultimately influencing how we understand and address mental health concerns and informing policy decisions and interventions.
Obtaining truly representative data samples in mental health research presents several challenges. If we don't carefully consider the demographics of the target population, certain groups might be overrepresented or underrepresented, significantly skewing the results. This can lead to prevalence rates that are off by a substantial margin, possibly as much as 30%.
To mitigate this, it's beneficial to employ a technique called stratified sampling. In this approach, we intentionally select subgroups within the broader population, making sure that characteristics like age or socioeconomic status are represented in the sample in the same proportion they appear in the larger population. This method helps enhance the accuracy of our prevalence estimates.
However, we should also be mindful of potential biases related to geography. Mental health data can often show a geographical skew, with urban areas potentially reporting higher prevalence rates due to increased stressors and access to mental health services. This may not accurately reflect the situation in more rural regions.
Another important consideration is cultural sensitivity. People's views on mental health can vary widely across different cultural backgrounds, which can influence their responses to surveys. If we don't adapt our survey instruments to be culturally appropriate, we risk introducing bias, leading to potential inaccuracies in prevalence estimates.
Non-response rates in surveys can be a major concern. Research has shown that people struggling with mental health issues are often less likely to participate in surveys. This can significantly bias our results, leading to an underestimation of true prevalence.
The rise of digital surveys has been transformative for research, but reliance on these technologies can inadvertently create biases in our data. Individuals who lack access to the internet or smartphones, particularly those from vulnerable populations, may be excluded from participation. This can produce samples that don't reflect the full diversity of the population.
Using snowball sampling, where participants refer others to the study, can be a way to access hard-to-reach groups, but it can also introduce bias. Participants might primarily refer individuals with similar characteristics, limiting the range of experiences captured in the sample.
Furthermore, major events like economic downturns or pandemics can significantly impact mental health prevalence. The timing of a study is therefore important. We must be aware of any significant events that could be influencing results.
It's also crucial to recognize that mental health conditions are not static. They can change over time in response to socioeconomic shifts, environmental stressors, or significant life events. This emphasizes the importance of conducting longitudinal studies to capture these dynamic aspects of mental health.
Finally, the use of incentives to encourage survey participation can impact the composition of the sample. Incentives may attract individuals who don't represent the typical population, potentially leading to skewed results and an inaccurate representation of true prevalence rates.
Calculating Mental Health Prevalence A 7-Step Guide for Accurate Assessment - Analyzing Data and Identifying Trends
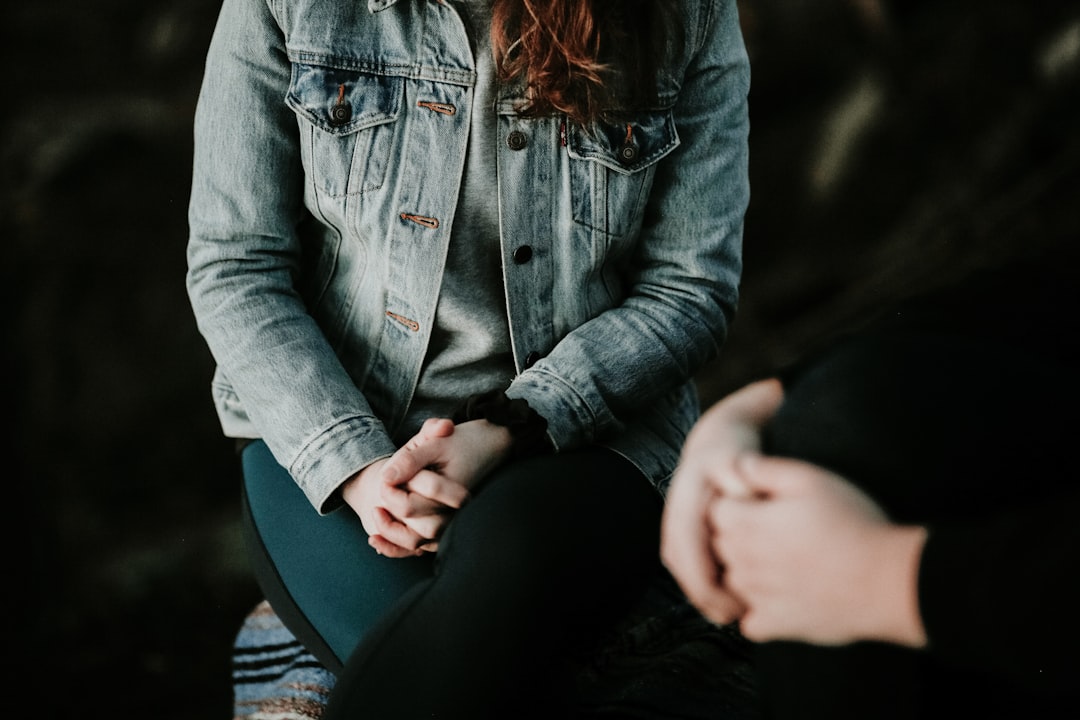
Understanding the changing patterns of mental health is crucial, and analyzing data to uncover trends is a key part of this process. We've seen a clear rise in the prevalence of mental health conditions in recent times, especially after the COVID-19 pandemic, with anxiety and depression being particularly prominent across various population segments. Researchers are using methods like predictive analytics and more sophisticated sampling techniques to make sense of the data gathered from different sources. However, it's crucial to be aware that the process of gathering and interpreting data can be susceptible to bias, which might result in skewed views of mental health prevalence, particularly when it comes to groups that are underrepresented in research. As we improve how we analyze data, it's vital that we understand the influence of cultural background, economic circumstances, and location to more accurately reflect the full spectrum of mental health issues and needs within the population.
Studies show a substantial increase in common mental health conditions from 2007 to 2019, coinciding with a rise in the use of both general medical and specialized mental health services. This pattern is reflected in the NEMESIS studies, which highlight a growing demand for mental health care across different healthcare settings. Interestingly, algorithms are being developed that can predict mental health by analyzing online behavior, such as language and sentiment, while considering individual characteristics like demographics and cultural background.
The COVID-19 pandemic undeniably had a significant impact on mental health, particularly leading to increased anxiety and depressive disorders. Researchers are meticulously documenting this impact across different regions and age groups. On a broader scale, the Global Burden of Disease study has used data from various nations to estimate the prevalence of various mental illnesses, such as ADHD and substance use disorders.
A detailed review of prevalence studies has identified crucial factors influencing the quality of research, including the specific population and environment studied, how the condition is measured, and how statistical results are presented. These factors have been compiled into a 25-item checklist. Further, global research following the WHO's declaration of the COVID-19 pandemic indicates a sharp rise in mental health challenges, particularly anxiety and depression.
Systematic reviews of mental health and suicide have used pooled Odds Ratios to compare mental health disorder prevalence within affected groups versus control groups. The data collection process for prevalence studies often involves using software like Microsoft Excel and R to synthesize findings from extracted data. This points to a reliance on computation to make sense of sometimes messy data.
A major hurdle in mental health research is that many individuals requiring mental health services do not access them due to various obstacles, like a limited number of mental health professionals available. This disconnect between need and access presents a clear challenge in creating a more accurate picture of the true burden of mental health disorders in the population.
While it's clear that some progress is being made in understanding mental health through data and trend analysis, we must be very careful in how we interpret these findings. These issues are very complex and influenced by a wide range of human and societal factors that are difficult to measure accurately. There is no single cause or single solution, making it challenging to create universally applicable solutions. There's always a danger of oversimplifying a complicated issue which is likely to lead to even more issues than it solves. Furthermore, there are potential problems with bias and accuracy built into the methods, some of which have been mentioned. It's exciting that more complex statistical methods are becoming available, but it's vital that we are cautious of their results and also keep them grounded in the reality of the complexities of human behavior.
Calculating Mental Health Prevalence A 7-Step Guide for Accurate Assessment - Accounting for Demographic Factors
When calculating mental health prevalence, it's vital to consider how demographic factors influence mental well-being. Things like socioeconomic status, ethnicity, gender, and where someone lives can significantly impact their risk of developing mental health challenges. Research suggests that these demographic aspects can account for a large percentage of mental health issues, emphasizing the need for more targeted approaches to care and support. It's becoming increasingly clear that a one-size-fits-all approach to mental health interventions simply won't work due to the vast differences in how individuals experience mental health struggles.
The COVID-19 pandemic has brought this issue to the forefront, highlighting the need to identify and address the unique needs of particular demographic groups who may be more vulnerable to mental health problems. Accurate assessment is impossible without factoring in these demographic differences. Understanding these distinctions is crucial for developing effective strategies and allocating resources in a way that ensures everyone has access to the mental health support they need. Ultimately, acknowledging the impact of demographic variables on mental health allows us to build a more nuanced and accurate picture of prevalence, which in turn leads to better interventions and outcomes.
Mental health isn't uniformly distributed across populations. We see significant differences in the prevalence of mental health conditions based on factors like age, gender, and ethnicity. For instance, certain racial or ethnic groups might experience different rates of mental health diagnoses, highlighting the importance of understanding these variations when estimating prevalence.
The stage of life someone is in seems to play a big role in their vulnerability to certain mental health issues. Younger adults might experience higher anxiety during major life transitions, whereas older adults might be more susceptible to depression due to unique challenges associated with aging. This suggests a need for assessments tailored to different age groups.
Socioeconomic factors also play a substantial role. People from lower socioeconomic backgrounds often experience heightened stress, leading to increased prevalence rates of issues like anxiety and depression. This emphasizes the need for mental health research that considers financial circumstances.
There's a notable difference in mental health prevalence between urban and rural areas. Urban environments tend to report higher rates, potentially due to increased stress and easier access to services. It's important to recognize this disparity when interpreting findings and designing research.
Cultural differences significantly impact how people view and respond to mental health issues. The way a culture perceives mental illness influences how readily people seek help or disclose their experiences, which can distort prevalence estimations if not considered in the research design.
Our reliance on digital tools for data collection is a mixed bag. While these technologies offer broader reach, they can unintentionally exclude populations with limited internet access or digital literacy. This potential bias can skew results, potentially misrepresenting the prevalence of mental health challenges in marginalized communities.
One of the major hurdles in mental health research is the issue of non-response bias. People struggling with severe mental health concerns are often less inclined to participate in surveys, causing an underrepresentation of these individuals in the data. This makes it difficult to get a true sense of the prevalence rates for these specific populations.
Mental health conditions aren't static. They fluctuate over time, and things like economic downturns or public health events can play a big part in these changes. This underscores the need for ongoing longitudinal studies to monitor mental health trends and adapt our understanding of them.
The way we select participants for a study—the sampling technique—can heavily influence the accuracy of prevalence estimates. Strategies like stratified sampling can help create more representative samples that accurately reflect the different subgroups within a population, ultimately reducing bias.
Stigma surrounding mental health, often influenced by cultural and demographic factors, can make people reluctant to seek help or disclose their struggles. This stigma can significantly distort prevalence data as individuals might underreport their experiences due to fear of judgment or discrimination.
Calculating Mental Health Prevalence A 7-Step Guide for Accurate Assessment - Considering Environmental and Societal Influences
When determining the prevalence of mental health conditions, it's crucial to acknowledge the wide range of environmental and social factors that can significantly impact mental health. Things like the physical environment—including community design, access to nature, air quality, and the quality of housing—have been shown to influence mental well-being. Moreover, a growing body of research highlights the connection between mental health and broader social factors, like socioeconomic circumstances and cultural perspectives. This emphasizes that mental health isn't just an individual concern but is intricately woven into the fabric of society. Understanding these influences is vital as they not only shape individual experiences but also point to the gaps in our current understanding of mental health prevalence. It's crucial to thoughtfully consider these external factors when developing strategies to assess and intervene in mental health challenges, as a more holistic approach is necessary for more effective outcomes. Failing to do so can lead to misinterpretations of mental health patterns and a failure to create useful solutions.
Mental health prevalence isn't just about individual traits; it's intricately woven with the environments and societies we inhabit. This realization is changing how we assess mental health, pushing us to consider a broader range of factors. For example, the design of our cities—how much green space there is, how easy it is to walk around, and the quality of housing—can significantly influence stress levels and, therefore, mental health outcomes. We're also seeing how deeply embedded cultural perspectives on mental health are. Some cultures are more likely to stigmatize mental health issues, causing people to hide their struggles and leading to inaccurate prevalence data.
Social support networks also seem to play a huge role in mental resilience. People with strong social connections often show better mental health, indicating that societal structures and connections are key aspects in mental health prevalence. When looking at history, we can see that major economic downturns often lead to a significant rise in mental health disorders. Times of high unemployment and financial instability create increased anxiety and depression. Conversely, policies focused on improving mental health services can directly lead to changes in how often mental health issues are reported. This points to how public health interventions have the potential to shape mental health outcomes.
However, geographical differences often complicate this picture. Rural communities might face limited access to services but often report lower rates of mental health disorders than urban areas. This could be a reflection of limited access to services or a difference in the way mental health is addressed. It's crucial to acknowledge that major life events like starting college, becoming a parent, or entering retirement can significantly impact our vulnerability to mental health conditions. This emphasizes the need for more precise assessments that acknowledge these individual life stages.
The digital revolution has brought many positive developments to mental health, but it has introduced some new biases. Those without reliable internet access or those who are less comfortable with technology can easily be excluded from surveys. This potentially leads to biased prevalence estimates that only represent a segment of the population. Even if we don't want to focus on climate change itself, seasonal changes clearly impact mood disorders. This shows how natural cycles and environmental changes can influence mental health.
Finally, there is an emerging understanding that experiences of microaggressions and discrimination can have a cumulative negative effect on mental health, especially in marginalized communities. These daily encounters with subtle forms of bias and prejudice often lead to a chronic stress response, increasing the likelihood of anxiety and depression, issues that are often missed in prevalence studies. It's become clear that these environmental and societal factors are vital to accurately understanding and addressing mental health challenges. This emerging knowledge is helping to shape a more holistic understanding of mental health prevalence and will hopefully help lead to more equitable and effective interventions.
Calculating Mental Health Prevalence A 7-Step Guide for Accurate Assessment - Validating Results Through Peer Review
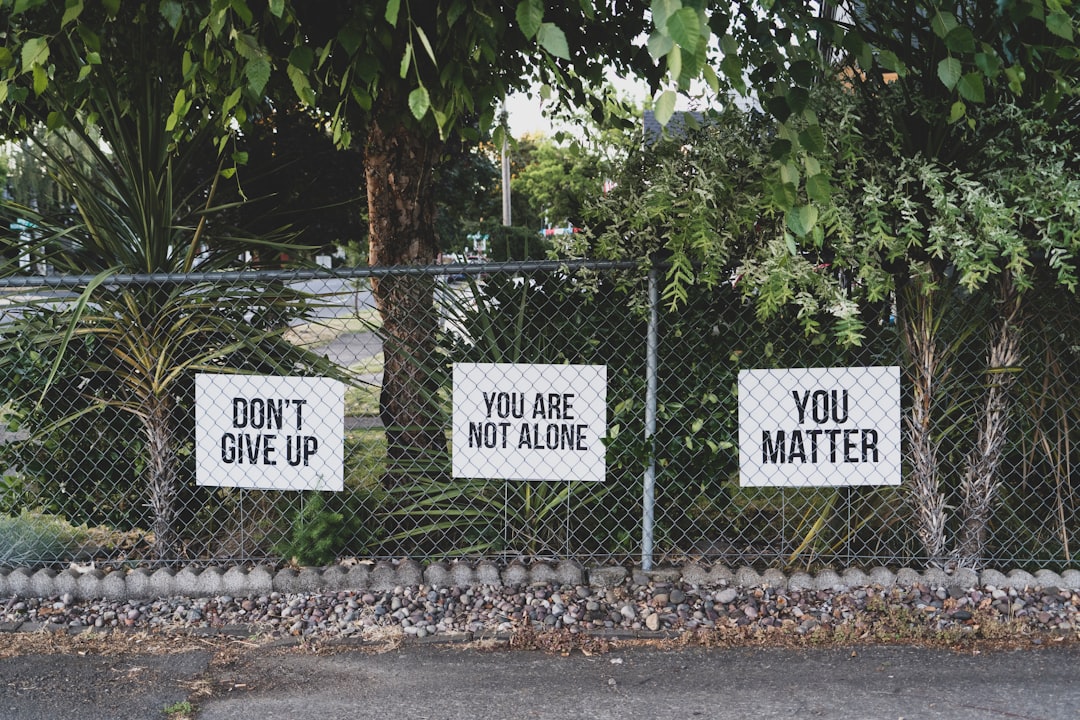
Ensuring the accuracy of mental health prevalence studies relies heavily on validating the results through peer review, a cornerstone of rigorous research. Peer review involves having experts in the field examine the research methods, data analysis, and conclusions of a study. This process is essential for identifying potential biases, weaknesses in the study design, or gaps in the data that might skew prevalence estimates. By scrutinizing the study, peer reviewers contribute to a more accurate and reliable understanding of mental health trends and patterns. This collaboration not only enhances the credibility of the research but also paves the way for methodological improvements in the development of validated assessment tools for future studies. Ultimately, comprehensive peer review is crucial for building a foundation of trustworthy evidence that can guide effective mental health interventions.
Validating results through peer review is a crucial step in mental health research, acting as a quality control mechanism before findings are made public. It helps prevent the spread of unreliable research that could distort our understanding of mental health prevalence, ensuring the scientific basis for our knowledge remains sound.
Evidence suggests that peer review enhances the overall quality of research, with reviewed studies often exhibiting stronger methodologies. This implies that feedback from peers can refine the tools used and the interpretations of data that ultimately influence how we perceive mental health prevalence.
However, the peer review process is not without its flaws. Personal biases can creep in, with reviewers potentially favoring research that aligns with their own views. This can unintentionally lead to certain populations or research outcomes receiving preferential treatment, thus skewing our collective understanding of mental health conditions.
A common issue in scientific fields, including mental health research, is a bias towards publishing studies with positive results. This tendency, known as publication bias, can lead to underrepresentation of negative or inconclusive findings, complicating the picture of accurate mental health prevalence even further.
Having diverse perspectives among peer reviewers is a powerful way to fortify a study, as different viewpoints play a vital role in identifying potential shortcomings or concealed biases. Studies that incorporate reviewers with a variety of backgrounds and expertise are more likely to produce thorough assessments of mental health prevalence.
Anonymity in the peer review process can sometimes hinder reviewers' willingness to provide truly critical feedback. While protecting the authors, it can also potentially weaken the quality of research outcomes. Encouraging more open feedback could lead to more rigorous examinations of the methodologies used to evaluate mental health prevalence.
The length of time it takes for the peer review process to complete varies considerably across different disciplines, and mental health research can be especially slow. These delays can impede the timely dissemination of important findings, such as shifts in mental health prevalence following significant events like economic recessions or major health emergencies.
Some argue that the established peer review system might unintentionally favor researchers who are already well-known over newer researchers. This can potentially stifle novel ideas, hindering the development of innovative assessment techniques that could better capture contemporary mental health trends.
The adoption of open peer review models, where reviewers are publicly identified, is a growing trend. This shift seeks to improve transparency and accountability in the review process, potentially leading to more insightful feedback, ultimately enriching our grasp of mental health prevalence.
Lastly, the rigorous nature of the peer review process can present obstacles for researchers, especially those from underrepresented groups. These barriers can reinforce inequities in mental health research, further hindering our efforts to understand and address prevalence within various population segments.
AI-Powered Psychological Profiling - Gain Deep Insights into Personalities and Behaviors. (Get started for free)
More Posts from psychprofile.io: