Navigating APA Ethics Code Citations A Practical Guide for AI Researchers in 2024
Navigating APA Ethics Code Citations A Practical Guide for AI Researchers in 2024 - Understanding the APA Ethics Code in the Context of AI Research
The APA Ethics Code, while foundational for psychological research, faces a new test in the era of AI. The rapid advancement of AI technologies, particularly generative AI and large language models, has outpaced the development of clear ethical guidelines specifically tailored to this domain. While the core principles of the code remain relevant, the unique complexities of AI necessitate a careful reevaluation and potential adaptation of existing norms.
AI research, though promising in its potential to enhance research speed and accuracy, brings with it a range of ethical quandaries that need to be addressed in a thoughtful and comprehensive manner. These include considerations like the societal implications of AI, the crucial role of human oversight and judgment in AI-driven decisions, and the need for researchers to approach ethical reasoning in a dynamic and evolving context.
Moreover, the landscape is characterized by a ‘Triple Too’ issue: an abundance of broad ethical initiatives, abstract principles that lack practical application, and an undue emphasis on the potential risks of AI versus its potential benefits. The need for specific guidance, beyond the existing frameworks, is evident, as current literature suggests a deficit in dedicated AI research ethics guidelines. Adapting existing processes, such as the role and practices of Institutional Review Boards (IRBs) in assessing AI-driven research projects, will be essential.
The integration of AI in psychological research demands a fostering of ethical awareness across the field. Researchers must be equipped to navigate the ethical complexities inherent in AI research responsibly, ensuring a balance between leveraging AI's potential and mitigating potential harm, fostering a culture where responsible AI integration becomes the norm.
The APA's ethical guidelines, though foundational for psychological research, present a unique set of considerations when applied to the field of AI. Transparency, a core principle in the APA code, is particularly vital in AI research, as the complex nature of these systems requires researchers to be explicit about their methodologies and findings to ensure others can scrutinize and potentially replicate their work.
Applying the concept of beneficence in AI is especially tricky. The potential impacts of AI can be hard to foresee, making it difficult to fully gauge the balance between advantages and potential harm. The need for informed consent becomes complex when dealing with AI, especially in cases where individual participant data is less clear-cut or anonymized.
Furthermore, protecting participant confidentiality in the age of large datasets presents a significant challenge. The APA's emphasis on confidentiality necessitates careful attention to data security, as even seemingly anonymized information can potentially reveal personal details. The principle of justice brings into sharp relief the complexities of equity in AI research. Ensuring that the benefits and risks of AI technologies are fairly distributed, especially among historically underrepresented communities, is a critical concern.
The APA emphasizes avoiding harm, yet the capacity for AI to perpetuate existing societal biases creates a potential for unintentional damage to vulnerable populations. While informed consent is fundamental, it becomes more nuanced in the dynamic world of AI. Researchers must carefully navigate conveying to participants the full scope of how their data might be used across the entire research process, especially given the evolving nature of AI systems.
Continuing education and training are vital for researchers operating within the ever-changing landscape of AI. The APA code underscores the importance of staying abreast of new ethical and technological developments as part of a researcher's ongoing commitment to responsible practices. The tension between commercial incentives and the ethical obligation to prioritize participant welfare and scientific integrity often gives rise to difficult dilemmas.
The call for accountability in the APA Code urges AI researchers to look beyond traditional research boundaries and consider the wider societal implications of their work. This means anticipating the potential impact of AI technologies on society, encouraging researchers to take a more proactive role in ensuring their work is used in a way that benefits humanity while mitigating potential negative consequences. This necessitates a shift in mindset and a greater level of foresight in the field of AI development and implementation.
Navigating APA Ethics Code Citations A Practical Guide for AI Researchers in 2024 - Key Ethical Principles for AI Researchers in 2024
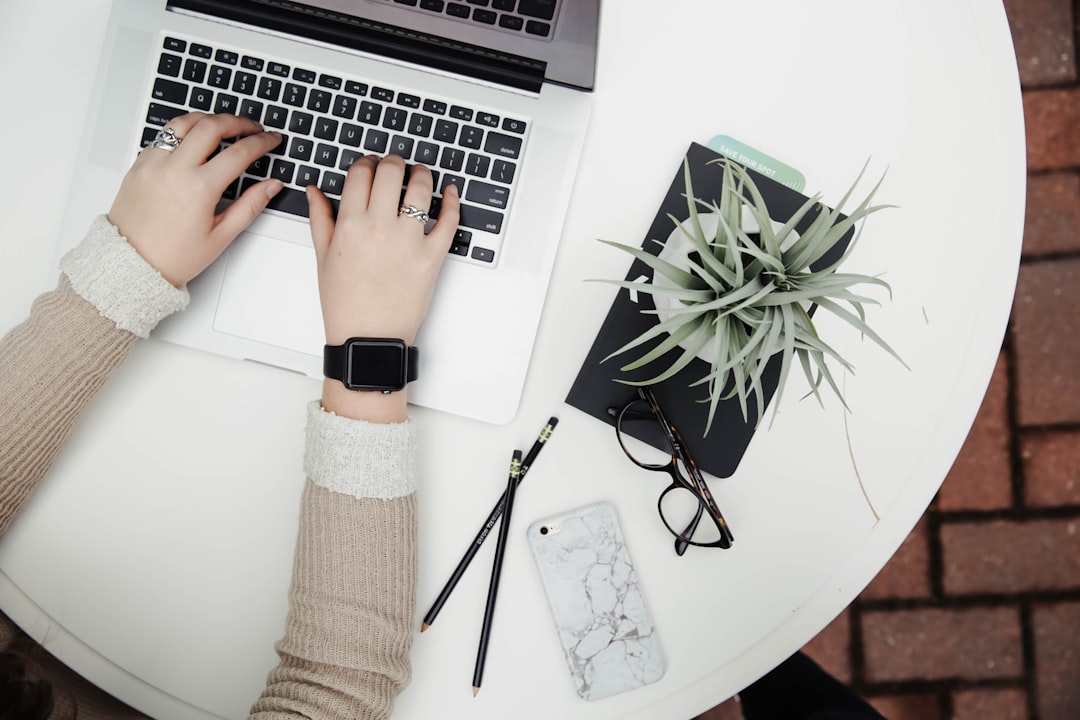
The field of AI research in 2024 is marked by a rapid pace of technological advancement, raising critical ethical concerns. Researchers must prioritize transparency in their work, especially given the complex nature of AI systems. This involves clearly documenting methods and findings to allow for scrutiny and potential replication by others. Furthermore, the principle of beneficence takes on new dimensions in AI, as researchers grapple with the challenge of anticipating and balancing potential benefits against unforeseen harms. This necessitates careful consideration of the impact on society, including the potential for exacerbating existing biases and inequalities.
The question of justice within AI research becomes increasingly important, particularly the need to ensure that advancements are distributed equitably across various populations. AI has the potential to amplify societal biases, so researchers must be vigilant in mitigating potential harm to vulnerable groups. Traditional ethical considerations, such as informed consent, are also re-evaluated in the AI context. Researchers need to be more proactive in educating participants about how their data may be utilized throughout the research process, especially given the ever-changing nature of AI applications.
The ethical landscape of AI is constantly shifting, necessitating continuous learning for researchers. Maintaining awareness of emerging ethical and technological developments is crucial. There is a growing call for clear, actionable guidelines specifically tailored to the unique ethical challenges of AI. This need for tailored guidance is becoming more urgent as the integration of AI into everyday life increases. Moreover, researchers are being urged to look beyond the immediate scope of their projects, taking a broader view of the societal implications of their work. This responsibility extends to proactively anticipating and mitigating potential risks to society and fostering a future where AI serves humanity in a beneficial and equitable manner.
The accelerating development of AI has brought into sharp focus the ethical complexities surrounding algorithmic biases. Researchers need to go beyond simply understanding the mechanics of AI and actively seek out and remove biases embedded in data, as these can lead to unfair or discriminatory outcomes.
The traditional notion of informed consent is facing a reimagining within AI research. It's not enough to explain what data will be collected; researchers must also clarify how generative processes might reprocess and repurpose that data in ways that might not have been anticipated, leading to a need for a more nuanced approach to consent.
Transparency, while a cornerstone of ethical practice, is challenging in the intricate realm of AI systems. Their inner workings are often veiled, making it difficult for outside researchers to evaluate research methodology and reproduce results, potentially hindering proper ethical scrutiny.
The fast-paced evolution of AI technology creates an ongoing ethical challenge in terms of accountability. As AI systems are continuously updated, so are the potential ramifications of research outputs. Researchers are forced to remain alert to these dynamic shifts and adapt their understanding of their ethical duties.
Protecting data privacy in the age of AI amplifies existing worries about confidentiality. Researchers must take stronger security precautions to safeguard not only obvious personal information but also data that could be used to indirectly identify individuals through data mining techniques.
Applying the concept of beneficence in AI research is further convoluted by the unpredictable nature of machine learning models. Benefits can quickly be outweighed by unanticipated harm, especially in sensitive fields such as healthcare or the legal system.
IRBs are facing the difficult task of adapting to the rapid advancements in AI. This emphasizes the urgent need for updated guidelines to address the distinct ethical challenges that come with AI-driven research.
Fairness in AI research isn't just a moral issue, it's a vital aspect of scientific integrity. Ensuring a variety of research participants is key to reducing the possibility of harmful applications affecting already disadvantaged groups.
Continuous education for AI researchers isn't just beneficial, it's an absolute necessity. A lack of knowledge about the constantly changing technological landscape can easily lead to major ethical transgressions and damage public trust.
The capacity of AI systems to unintentionally contribute to the spread of misinformation creates fresh ethical responsibilities for researchers. They need to integrate the concepts of accuracy and truthfulness into the core values of their research.
Navigating APA Ethics Code Citations A Practical Guide for AI Researchers in 2024 - Proper Citation Methods for AI-Generated Content
The growing use of AI in research necessitates clear guidelines for citing AI-generated content. Researchers are now expected to be transparent about the role of AI in their work, including the specific AI tools and prompts utilized. While APA style calls for crediting the AI tool as the author, it's important to understand that the output from these tools is considered non-retrievable data, similar to how personal communications are cited. This means specific details of the AI's role in generating data or text, including prompts and methods, are important. Maintaining transparency is crucial for upholding accountability and ethical considerations. Properly citing AI-generated content ensures others can understand the research process and evaluate the reliability of findings, allowing for effective scrutiny and replication within the research community. Researchers must strike a balance between utilizing AI effectively and adhering to the ethical principles of the APA to navigate the ethical landscape of AI integration in research.
1. **Adapting Citation Practices**: We're seeing a growing need to adapt how we cite AI-generated content in 2024. Traditional methods aren't always sufficient when trying to capture the role of these complex systems in our research. It's raising interesting questions about authorship and responsibility, which is prompting discussion.
2. **AI in the Author Spot?**: Some publications are actually starting to list AI systems as co-authors, which is a pretty significant change. It suggests that some people are beginning to view AI as having a level of intellectual contribution. This necessitates a fresh approach to clarify how AI fits into the research process and the creation of research outputs.
3. **The Source of the Source**: Proper citation for AI content is pushing us to really consider the 'source' of the training data. It's making researchers more aware of the need to be transparent about the datasets used and to be responsible about how they're handled. This aspect of accountability is increasingly important.
4. **Citation Formatting Gets Tricky**: The complexities of AI-generated work are making traditional citation formats feel a little clunky. The iterative nature of these models, and how they arrive at outputs, doesn't neatly fit into existing methods. It suggests we may need to refine our standards for clarity.
5. **Keeping Track of Versions**: Given how AI systems can change over time, it makes sense to start including the specific version of the AI model used in a citation. Similar to how we version software, this helps ensure reproducibility and adds another layer of transparency to the research process.
6. **The Ghost of Bias**: We have to be mindful of biases within AI training data that could influence the output. This calls for more critical evaluation of the results AI produces and requires us to be honest about the possibility of bias in our citations.
7. **Informed Consent in the AI Age**: When citing AI tools, it's important to disclose how the content was generated. However, this raises ethical questions about informed consent, particularly if participants aren't aware their data might contribute to AI training.
8. **Bridging Disciplines**: AI research crosses many fields – computer science, ethics, psychology – and the need for good citations has to reflect that. This multidisciplinary nature adds complexities to our existing expectations of what constitutes proper citation across the spectrum.
9. **Regulations on Citations**: It's encouraging to see that governing bodies are developing guidelines for citing AI-generated research. It shows a growing understanding that we need a specific ethical framework for research that uses technology in these novel ways.
10. **Maintaining Research Integrity**: If we're not clear and honest about the role of AI in our research, it can mislead readers and damage the integrity of the research itself. It's a call for more precise standards in this area to foster accountability and transparency in AI-driven research.
Navigating APA Ethics Code Citations A Practical Guide for AI Researchers in 2024 - Balancing AI Benefits and Ethical Concerns in Research
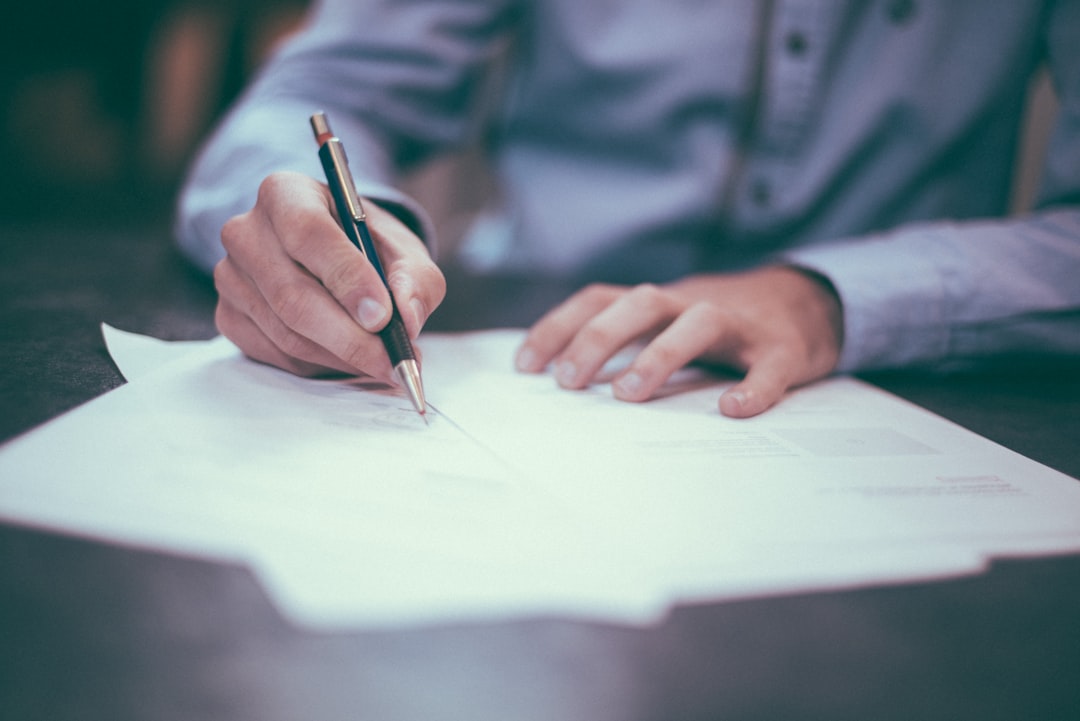
The integration of AI into research presents a complex landscape where the potential benefits must be carefully weighed against significant ethical considerations. While AI offers promising avenues for advancing research, it also introduces a unique set of challenges that necessitate new ethical guidelines. The rapid pace of development in this field demands ongoing scrutiny of the methods, assumptions, and potential impacts of AI on society. This requires researchers to adopt a reflexive approach, constantly evaluating their work in a broader context. Furthermore, researchers need access to appropriate ethical training so that they can responsibly integrate AI tools into their research practices, ensuring innovation is always guided by ethical considerations. The dynamic nature of AI technologies necessitates a flexible approach to ethical decision-making, highlighting the urgent need for researchers to proactively address the multifaceted ethical implications of AI-driven research.
1. **Setting New Ethical Standards:** The way AI is used in research could establish new ethical norms, much like the need for researchers in biomedical fields to declare potential conflicts of interest. This signifies a potential shift towards greater openness and transparency within scientific publications.
2. **How Participants View Their Role:** When AI is involved, participants might have a different perception of their involvement in a study. The technical complexity might cause them to underestimate or misinterpret the impact of their contribution, which makes gaining informed consent more challenging.
3. **Ethical Guidelines in Flux:** The ethical guidelines around using AI aren't fixed; they're constantly changing as researchers grapple with the effects of their work on society. This means that ethical codes need to be regularly updated to remain relevant.
4. **Checking for Bias in AI**: A new trend in AI ethics is doing 'algorithmic audits', where researchers carefully examine AI systems for bias and fairness before they're used. This recognizes that the results of AI can reinforce existing inequalities.
5. **Researchers' Responsibility for AI Outputs:** Even when AI systems automate some tasks, researchers are still the ones responsible for making sure AI-generated outputs are used ethically. The human researchers who guide the research ultimately remain accountable.
6. **Where Data Comes From Matters**: With AI models, there's increasing focus on 'data provenance' – tracking where the training data originates. This underscores the importance of recognizing how biases can spread through the data that AI systems learn from.
7. **Working Together Across Disciplines:** Addressing the ethical complexities of AI research often calls for a team effort that includes tech experts, ethicists, and social scientists. This is because AI systems have implications that stretch across many areas of knowledge.
8. **Learning from Past Mistakes:** Past cases of unethical research (like the Tuskegee Study) remind us of the importance of avoiding ethical missteps, especially when it comes to protecting vulnerable populations in AI research.
9. **Rethinking Informed Consent:** As AI's capabilities increase, traditional ways of getting informed consent are being questioned. We need new approaches to make sure participants truly understand how their information could be used in ways they might not anticipate.
10. **Maintaining Public Trust in Science:** If AI is misused in research, it could damage public trust in science. Researchers need to be very clear about the ethical factors involved in their work and explain their methods to maintain credibility and keep society's support for science.
Navigating APA Ethics Code Citations A Practical Guide for AI Researchers in 2024 - Implementing Transparency in AI-Assisted Studies
The increasing use of AI in research necessitates a strong emphasis on transparency. As AI systems become more sophisticated and integral to research processes, researchers must make their methods and results readily understandable to others. This ensures that the work can be carefully examined and potentially replicated, which are foundational elements of good research practice. However, the rapid development of AI has also brought into focus a need to reassess how we apply traditional ethical standards, particularly when it comes to how we obtain informed consent from participants and safeguard their privacy. The ever-expanding potential of AI in research also raises critical questions around fairness and access, highlighting the ethical burden researchers have to address potential biases that might disadvantage marginalized communities. It's clear that establishing an environment where transparency and accountability are central to research practice will be crucial for addressing the specific challenges that AI poses in the field.
The rapid integration of AI, particularly large language models, into research has outpaced the development of ethical guidelines, specifically concerning transparency. Transparency in AI-assisted research now goes beyond simply describing methods; researchers need to clarify the complex processes and algorithms used to generate and utilize data, adding a new layer of complexity to ethical practices and making reproducibility a tougher challenge.
As AI research enters the public eye, there's a rising call for clear communication and accountability. Researchers are being pushed to better align their research with the values of society, engaging with diverse stakeholders who can shape ethical standards.
Unfortunately, AI model outputs often aren't easily explained, which can hinder a researcher's ability to clearly describe their methods. This lack of clarity could interfere with effective peer review and ethical oversight, leading to questions about the overall rigor of a study.
It's becoming apparent that different fields are adopting distinct approaches to incorporating transparency into AI-assisted studies, resulting in conflicting standards that could complicate interdisciplinary collaboration and the comparison of research results.
In studies employing generative AI, tracking the origin and usage of training data is challenging. This can obscure who is responsible for biases or mistakes in the research findings, potentially undermining core ethical principles.
To increase transparency, some researchers are implementing regular audits of AI systems to identify biases and ethical concerns as they evolve, seeking a more continuous approach to maintaining integrity.
In the AI age, traditional informed consent processes are under scrutiny. Researchers face the challenge of making sure participants fully grasp where their data is headed and how it may be used across the ever-changing realm of AI.
Successfully implementing transparency in AI demands a range of expertise, forcing researchers to work alongside engineers, ethicists, and other specialists. While this cross-disciplinary approach might be more intricate, it also encourages a richer ethical dialogue.
Researchers are starting to quantify the effects of algorithmic bias in their studies. They're discovering that failing to address these biases not only raises ethical issues, but can also result in flawed interpretations of data.
Because of AI's contribution to the spread of misinformation, researchers need to take a more active role in presenting their findings accurately and transparently. Transparency is increasingly recognized as a critical defense against unethical practices within research.
Navigating APA Ethics Code Citations A Practical Guide for AI Researchers in 2024 - Adapting Traditional Research Ethics to AI-Driven Methodologies
The increasing use of AI in research necessitates a careful rethinking of traditional research ethics. The rapid pace of AI development has outstripped existing ethical guidelines, leading to a need for researchers to navigate both the potential advantages and the risks inherent in AI-powered research. A major issue is the disconnect between broad ethical principles and their practical application in the real world of AI. We urgently need more specific guidance that can tackle the complex ethical problems presented by AI in various research contexts. Furthermore, issues such as informed consent, the protection of data privacy, and the detection and mitigation of algorithmic biases need careful examination to ensure fairness and retain public confidence in research. Addressing these evolving ethical complexities requires a commitment to ongoing ethical reflection and adaptation within the research community, fostering an environment where AI is integrated into research practices in a responsible and ethical manner.
The rapid rise of AI, especially large language models, has outpaced the development of ethical guidelines specifically designed for its use in research. A recent study found that a small fraction of research articles about AI in research ethics actually addressed the role of research ethics review boards, highlighting a knowledge gap in this area. One of the main hurdles in AI ethics is what some are calling the “Triple Too” problem: a plethora of high-level, often abstract ethical principles that lack practical applications and tend to focus more on the potential dangers than the possible upsides of AI in research.
While traditional ethical standards in research are still relevant, the unique aspects of AI research require new guidance and adaptation. There's a real difference between the theoretical discussions of AI in research ethics and the practical, real-world challenges we see in areas like healthcare. For instance, the increasing use of AI in research has raised questions about the validity and integrity of research publications. Many organizations are creating AI ethics guidelines built on a set of principles intended to manage the disruptive force of these new technologies.
Effectively implementing ethics review processes is essential for forecasting and minimizing potential harm in AI research. Because of AI's role in scientific research, we need to reconsider the current ethical frameworks to tackle brand-new ethical questions. There's a call for scientists to develop AI ethics guidelines that are specific to certain contexts and that can bridge the space between broad principles and their real-world application. Essentially, the practical application of AI research ethics is lagging behind AI’s rapid development, forcing us to rethink how we conduct research while upholding ethical principles. The need for clear, context-specific guidelines is becoming more pressing as AI’s integration into various aspects of life, including research, accelerates.
More Posts from psychprofile.io: