New Neural Networks Reveal Hidden Biases A Deep Dive into AI-Powered Implicit Association Analysis
New Neural Networks Reveal Hidden Biases A Deep Dive into AI-Powered Implicit Association Analysis - Neural Networks Show Gender Bias in Medical Image Recognition Study at Stanford 2025
Recent findings emerging from a Stanford University study in 2025 have brought to light significant gender bias embedded within the neural networks utilized for interpreting medical images. This research suggests that despite the technical sophistication of algorithms now prevalent in medical analysis, they are susceptible to reflecting and potentially worsening societal disparities. The study highlighted instances where the accuracy of image interpretation appeared to vary based on gender, pointing to how imbalances or insufficient diversity in the data used to train these models can directly translate into biased outputs. This discovery necessitates a more rigorous examination of the data inputs and development processes for these AI systems, emphasizing that without actively addressing such hidden biases, the deployment of artificial intelligence in clinical settings risks perpetuating diagnostic inequalities rather than mitigating them. The complexity of these models further complicates efforts to understand and rectify these biases, urging a deeper dive into their operational flows.
The research conducted at Stanford in 2025 offered a stark look at gender-based biases embedded within neural networks utilized for analyzing medical imagery. The findings indicated a discernible disparity in diagnostic performance, where, on average, these AI models provided more accurate assessments for male patients compared to female patients. This difference was significantly linked to the composition of the datasets used for training; researchers observed that training corpora frequently contained a disproportionately higher number of images from male individuals, naturally leading to performance metrics that were skewed towards this demographic. This imbalance became particularly problematic when analyzing medical images from female patients, resulting in a higher propensity for misinterpretation. The study pointed out this challenge was especially pronounced in conditions where clinical presentation or radiographic appearance can vary between genders, such as certain cardiovascular ailments, often leading to an increased rate of false negatives for women. What was particularly noteworthy was the finding that while data imbalance was a primary driver, the architectural choices within some neural network designs themselves appeared to amplify these existing disparities, suggesting that careful model construction is as critical as data preparation. These revelations have understandably fueled conversations regarding the ethical considerations of integrating biased AI tools into healthcare, prompting essential questions about responsibility and the potential need for structured regulatory frameworks. Consequently, there's a growing push among researchers to curate and employ more diverse datasets that accurately mirror the demographic distributions relevant to various medical conditions, aiming to enhance the fairness and robustness of these models. The study also reinforced the critical need for transparency, emphasizing that medical professionals relying on AI assistance must be fully aware of the systems' limitations and inherent biases. Looking ahead, the team involved is reportedly investigating explainable AI methods as a potential avenue to decode the intricate decision-making pathways of these networks, which might offer ways to identify and possibly counteract these biases, perhaps even during clinical use.
New Neural Networks Reveal Hidden Biases A Deep Dive into AI-Powered Implicit Association Analysis - MIT Research Maps Racial Discrimination Patterns in Job Application AI
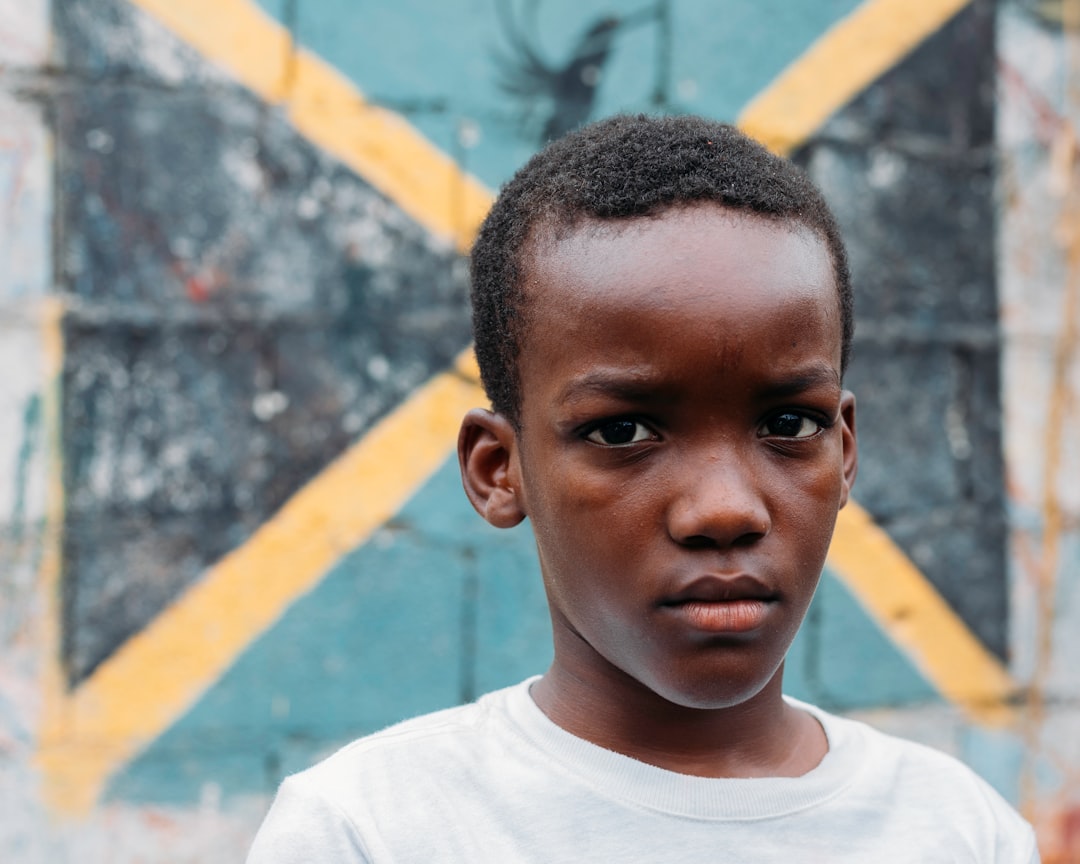
Recent findings from researchers at MIT have shone a light on how artificial intelligence systems used in hiring can embed and perpetuate patterns of racial discrimination. With AI now deeply integrated into the recruitment processes of many large companies, accounting for its use in screening by a vast majority, this research underscores a critical concern. The studies indicate that these tools often inherit biases from the data they are trained on or from the historical patterns they learn, leading them to disadvantage candidates from certain racial backgrounds. This can manifest in various ways, from how resumes are ranked to how applicants perform on AI-evaluated tasks or even how their names are perceived. While there is ongoing work, including some from MIT itself, exploring algorithms intended to increase diversity, the reality is that many widely deployed systems continue to reflect and scale existing societal inequities. Identifying these hidden discriminatory patterns is a necessary step towards understanding the challenge, but addressing the systemic issues enabling them within AI remains a significant hurdle requiring more than just technical fixes. The widespread reliance on these potentially biased tools necessitates careful scrutiny and a clear push for greater accountability in their design and deployment.
Research stemming from MIT has spotlighted disconcerting patterns of racial discrimination within AI systems leveraged for processing job applications. Given that various forms of automated hiring technology, including resume screens and profile analysis, are reportedly integrated into the processes of a significant majority of large corporations, this research feels particularly salient. The findings suggest that these tools are far from neutral arbiters of merit; instead, they appear capable of reflecting and even amplifying existing societal prejudices.
One notable observation highlighted by the research was the AI's propensity to evaluate candidates differently based on perceived ethnic cues, such as names, often favoring those traditionally associated with dominant groups. Beyond names, it was found that seemingly minor variations in a candidate's presentation or application format could have disproportionate negative effects depending on their racial background, indicating a sensitivity to surface-level attributes rather than a deep analysis of qualifications.
The core mechanism underpinning these issues often traces back to the data used to train these systems. If historical hiring data or the broader web data they learn from is skewed by past inequities or lacks diverse representation, the resulting AI models will inevitably absorb and replicate those biases. This isn't just a theoretical concern; other studies have documented specific instances of anti-Black bias in recruitment tools, ranging from profile analysis to more complex systems incorporating behavioral cues.
Critically, the research underscores that these AI systems are not merely passive tools. By internalizing historical data reflecting systemic biases, they risk actively participating in the perpetuation of discriminatory hiring practices at scale. The analysis implies that biases aren't static; they can potentially become more ingrained as systems continuously learn from biased streams of data. This necessitates a proactive approach, suggesting that transparency in how these algorithms function and regular, critical audits are crucial. Without robust accountability measures and a conscious effort to address the historical context embedded in training data, the widespread deployment of AI in hiring could inadvertently deepen existing divides rather than bridge them.
New Neural Networks Reveal Hidden Biases A Deep Dive into AI-Powered Implicit Association Analysis - Language Model Geography Test Reveals Western Knowledge Preference
Studies into language models recently underscore a distinct inclination towards information originating from Western geographies when assessed on their world knowledge. This observed preference suggests these artificial intelligence systems may hold a narrower perspective than assumed, potentially disseminating incomplete or disproportionate portrayals of places, societies, and events outside that sphere. Such an inherent tilt matters because it could subtly shape how individuals using these tools understand the global landscape. Efforts are underway to enrich the data these models learn from with more varied perspectives and develop methods to counteract these embedded tendencies, emphasizing the ongoing difficulty in achieving true equity in AI's representation of the world.
Observations from recent investigations into language models have brought to light a distinct unevenness in their comprehension of world geography. Specifically, it appears these models tend to exhibit a notable preference for information and perspectives originating from Western regions. This inclination seems rooted in the data they were trained on, potentially leading systems to foreground Western narratives in various applications, whether generating educational content or assisting with policy considerations.
Further scrutiny employing methods akin to implicit association analysis techniques suggests that this Western inclination isn't merely superficial. The underlying knowledge structures within these models sometimes struggle with accurately representing or interpreting regions with less extensive digital footprints or historical documentation, occasionally resulting in oversimplified or even misleading depictions of non-Western cultures and their associated geographies. This seems to create what some might term a "geographical blind spot," where the perceived significance of a location might align more with its prominence in Western media or scholarship than its actual global or historical importance, potentially overlooking crucial events or contributions from less-represented parts of the world. Questions naturally arise regarding the ethical responsibility of those developing these systems, particularly in ensuring a more balanced and equitable representation of global knowledge, pushing for more comprehensive and inclusive datasets to move towards AI tools that genuinely reflect diverse global perspectives.
New Neural Networks Reveal Hidden Biases A Deep Dive into AI-Powered Implicit Association Analysis - Age Related Decision Making Skew Found in Insurance Assessment Networks
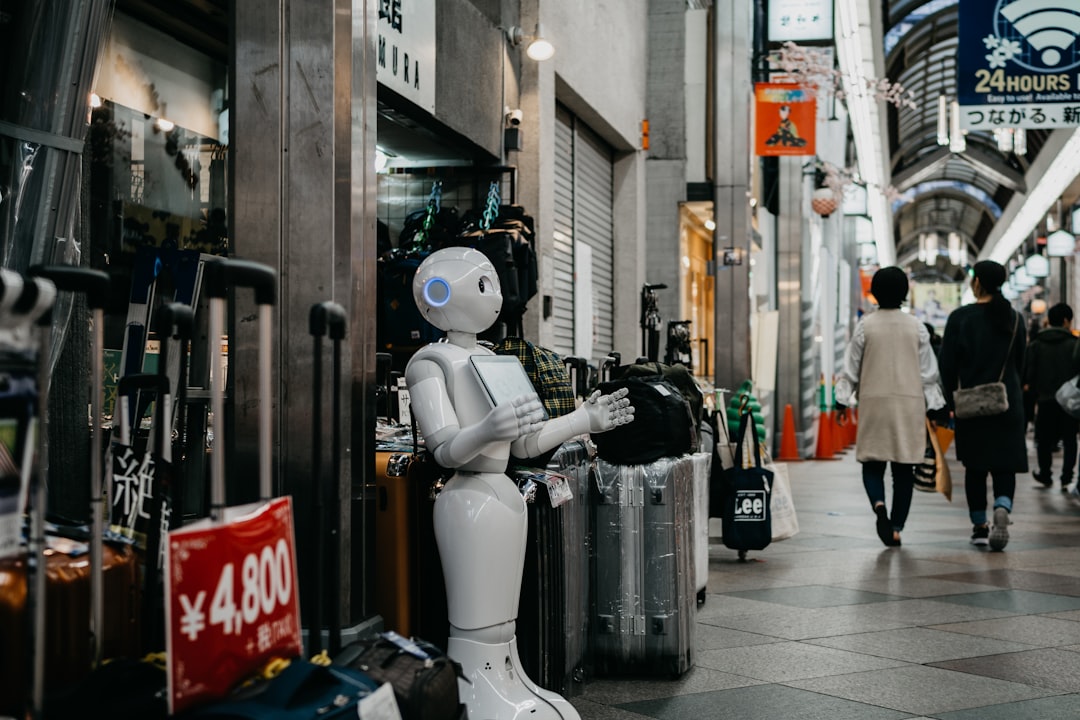
Within insurance assessment networks employing advanced AI, patterns indicating age-related skew in decision-making have emerged. These analyses, often powered by neural networks, appear to uncover hidden biases reflecting implicit associations connected to age groups. This phenomenon seems partly related to documented differences in how older individuals approach decisions, including distinct strategies for evaluating options, assessing risk, and processing information – often involving slower evidence accumulation. The presence of such embedded biases raises concerns about potentially inequitable treatment for older adults navigating insurance evaluations. Addressing how these age-related dynamics influence AI systems is crucial for developing fairer practices in this sector.
1. Observations suggest decision networks employed in insurance evaluation might carry biases linked to age, potentially leading to less favorable outcomes for older individuals, seemingly influenced by underlying assumptions about age and associated risk profiles rather than individual circumstances.
2. A contributing factor appears to be the uneven distribution within training data, where models often learn from a disproportionately smaller sample size of older demographics. This imbalance can negatively impact their ability to perform accurately and fairly when assessing this particular group.
3. It seems these automated systems may inadvertently embed implicit connections tied to age stereotypes, potentially steering decisions on claim approval or denial based more on demographic generalizations than a purely objective analysis of individual risk factors.
4. Some indications point towards potential delays in processing times for older claimants. This could stem from biases within automated systems that might implicitly prioritize or streamline workflows for younger applicants, inadvertently affecting timely access to necessary services for older adults.
5. The models might operate with a generalized view of risk that doesn't adequately capture the diverse realities and extensive life experiences of older adults. This can potentially result in broad, and at times inequitable, risk characterizations that do not reflect individual nuance.
6. A current challenge appears to be the relative lack of specific guidance within existing regulatory structures tailored to addressing age-related biases explicitly within AI systems used in sensitive domains like insurance, potentially leaving a gap in accountability for deployed algorithms.
7. The presence of these age-related patterns in insurance assessments certainly brings forward ethical questions regarding potential discrimination, where older individuals could face disadvantages not truly reflective of their personal risk but rather systemic biases embedded within the technology.
8. There's a clear and present need for greater transparency concerning the algorithms used in insurance evaluation processes. This is essential to facilitate independent review and help pinpoint, and subsequently address, any age-based biases that might be present and impacting outcomes.
9. With increasing public awareness regarding the potential for bias in AI-driven systems, there appears to be growing societal pressure on insurance providers to move towards more transparent and equitable practices that better reflect the actual diversity and complexity of the population they serve.
10. Continued investigation is essential to better understand the specific technical and data-driven mechanisms driving these age-related biases within neural networks, with an ongoing focus on developing technical interventions aimed at mitigating these effects and improving overall fairness in assessment processes.
More Posts from psychprofile.io: