Physiological Markers of Social Anxiety New Research Links Heart Rate Variability to SAD Severity (2025 Study)
Physiological Markers of Social Anxiety New Research Links Heart Rate Variability to SAD Severity (2025 Study) - Real-time HRV Monitoring Reveals Distinct Patterns During Social Interactions
Recent advances allow for real-time observation of physiological signals, specifically heart rate variability, during social interactions, revealing distinct patterns. This capacity enables researchers to capture how the body responds instantaneously to social cues. Evidence suggests that engaging in negative social exchanges is associated with a noticeable reduction in certain HRV markers. Interestingly, positive social interactions do not appear to elicit the same degree of physiological change, though higher baseline HRV has been linked to more effective or pleasant social engagements and potentially better emotional management, particularly in less intimate settings. While real-time monitoring and the use of wearable technology make it increasingly possible to track these physiological shifts over time and in varied social scenarios, interpreting the complexity of real-time autonomic responses within dynamic social contexts remains a considerable challenge. Nevertheless, these observable patterns offer potential insights into the physiological underpinnings of social behavior and may offer a useful window into how individuals, including those experiencing social anxiety, navigate their social world.
Leveraging real-time physiological monitoring is providing new avenues to explore how our bodies respond moment-by-moment during social engagements. Recent work using heart rate variability (HRV) measurements taken *as* interactions unfold is beginning to reveal distinct patterns that appear linked to an individual's emotional state and potentially, anxiety levels within that specific context. It’s less about a static marker and more about dynamic shifts.
The data emerging suggests that the emotional quality of a social interaction significantly impacts these real-time HRV patterns. For instance, studies indicate that exposure to negative social cues can prompt a noticeable drop in HRV – potentially reflecting a temporary shift towards sympathetic nervous system activation, the body's 'alert' state. Interestingly, positive interactions don't always elicit a mirroring increase in HRV, suggesting the autonomic response is not symmetrical and is particularly sensitive to perceived social threat or negativity. This dynamic change, or lack thereof, in HRV during an interaction seems to correlate with how comfortably individuals navigate these social spaces, aligning with the idea that vagally mediated HRV plays a role in our capacity for social engagement and regulating our responses in the moment. While promising, understanding the precise triggers and the clinical significance of these real-time shifts in diverse social scenarios remains an active area of investigation. Technology facilitating continuous, non-intrusive monitoring is certainly enabling larger datasets and more granular analysis of these complex physiological-social connections.
Physiological Markers of Social Anxiety New Research Links Heart Rate Variability to SAD Severity (2025 Study) - Parasympathetic Nervous System Activity Marks SAD Intensity in 240 Person Study
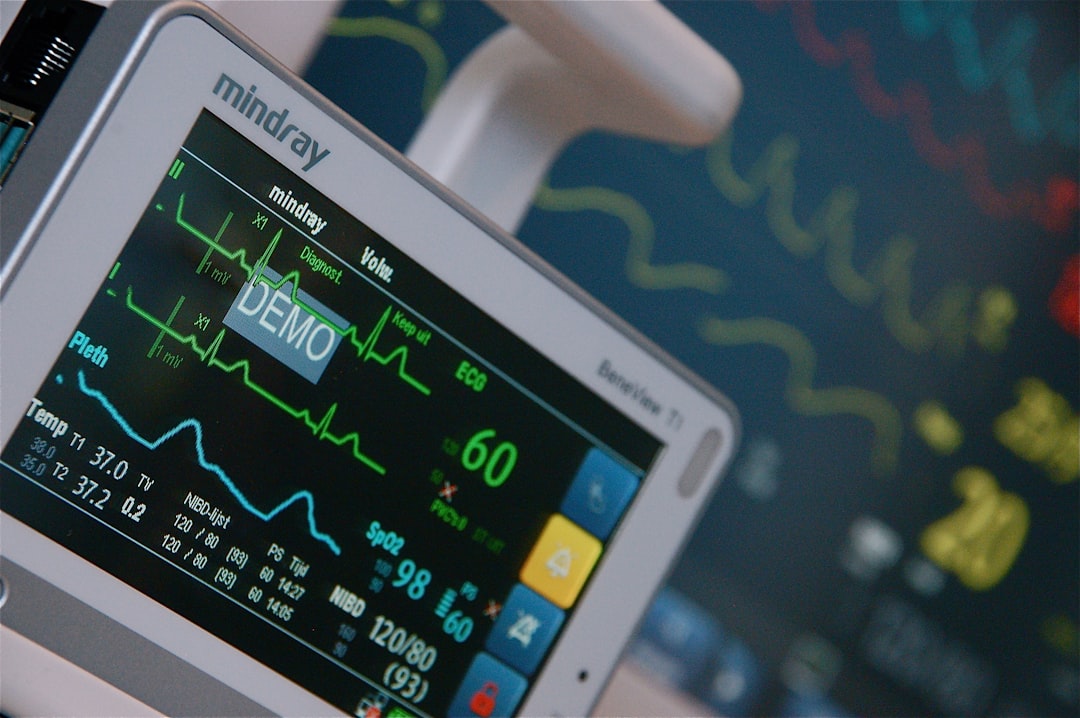
A recent study involving a substantial cohort of 240 individuals delved into the connection between activity in the parasympathetic nervous system (PNS) and the intensity of social anxiety disorder (SAD). This research found that measures of PNS function, notably heart rate variability (HRV), appeared associated with how severely social anxiety symptoms presented. Specifically, higher levels of baseline PNS activity, as indicated by greater HRV, tended to correlate with a lesser degree of reported social anxiety.
This observed link suggests that HRV might serve as a physiological marker reflecting the severity of the condition, pointing towards the parasympathetic system's fundamental role in regulating emotional and stress responses central to SAD. Furthermore, the study underscored the importance of not just overall activity, but also the *flexibility* with which the PNS can adapt its responses to various internal and external cues. Exploring this autonomic flexibility could offer deeper insights into the physiological underpinnings contributing to the challenges individuals with social anxiety face in managing distress. While these physiological indicators offer promising avenues for understanding the condition more objectively, integrating them into a comprehensive picture of social anxiety requires careful consideration.
A study involving 240 individuals has explored the connection between activity in the parasympathetic nervous system, often associated with states of rest and recovery, and the severity of social anxiety disorder (SAD). This research specifically utilized heart rate variability (HRV) as a window into parasympathetic function. Findings suggest that individuals who exhibit higher levels of parasympathetic activity, as reflected in their HRV patterns, tend to report less severe social anxiety symptoms. This points towards a potential physiological underpinning where a more robust parasympathetic system might be protective against the intensity of social anxiety.
Delving deeper, the study hints that the capacity of the parasympathetic system to flex and adapt, particularly the influence mediated by the vagus nerve, could be central to managing emotional and social challenges. While the preceding section discussed dynamic, real-time responses during interactions, this study adds a piece to the puzzle by showing this relationship in a larger cohort. For instance, measures like respiratory sinus arrhythmia were used to assess this flexibility in response to stimuli like sadness. The results suggest that individuals with seemingly greater "parasympathetic flexibility" demonstrated more pronounced withdrawal of vagal influence when exposed to such emotional cues, perhaps indicating a different regulatory strategy. However, translating the complex interplay of the autonomic nervous system, its inherent variability, and specific emotional regulation strategies in the context of SAD remains a significant analytical challenge. It’s clear that the biological markers associated with anxiety are intricate, and while studies like this enhance our ability to potentially phenotype these conditions more objectively, understanding the full picture requires accounting for this complexity.
Physiological Markers of Social Anxiety New Research Links Heart Rate Variability to SAD Severity (2025 Study) - Machine Learning Models Successfully Predict SAD Through Heart Rate Data
Recent developments suggest machine learning models are proving capable of predicting indicators of social anxiety by analyzing heart rate data, specifically focusing on heart rate variability (HRV). Taking advantage of increasingly common wearable sensors, various studies, including work published recently, are exploring how these algorithms can process physiological signals to potentially identify individuals experiencing higher levels of anxiety symptoms. This approach leverages the known link between HRV patterns and states of stress or anxiety. While different machine learning methods are being tested and refined, aiming to enhance assessment capabilities, it's crucial to acknowledge that translating these predictive insights into definitive clinical diagnosis is complex. The performance of these models can vary, and their training often relies on data labeled using traditional subjective measures. Despite these challenges, the capacity to extract meaningful physiological information like HRV from readily available cardiac monitoring opens a promising, non-intrusive avenue for potentially complementing existing methods used in understanding and monitoring social anxiety.
Drawing on advancements in machine learning applied to physiological monitoring, recent efforts aim to predict social anxiety disorder (SAD) severity using heart rate data, particularly heart rate variability (HRV). This line of research explores the potential for automated analysis of cardiac signals to support mental health assessment.
1. Certain machine learning models have demonstrated encouraging performance in distinguishing between different levels of SAD severity based on heart rate characteristics. Reports of accuracy metrics, sometimes exceeding 85% in specific classification tasks (like identifying individuals with high symptom load), suggest promise, although context and the specific criteria for defining 'severity' are critical details often needing closer scrutiny.
2. Analysis of these predictive models frequently highlights specific HRV features as particularly influential. Ratios and other metrics related to frequency domain analysis, often loosely linked to autonomic balance concepts like sympathetic and parasympathetic influence, tend to carry significant weight in the algorithms' decision-making processes, reflecting the presumed connection between autonomic regulation and anxiety states.
3. The ability of machine learning to process data streams continuously opens the door to utilizing the sort of real-time heart rate information increasingly available from wearable devices. The idea is that analyzing these signals as they occur could potentially offer more immediate insights into physiological responses associated with emotional states in relevant contexts, moving beyond static measures.
4. Some approaches attempt to build more comprehensive models by integrating data from multiple physiological sources beyond just heart rate, including signals like skin conductance or respiration. This multi-modal strategy seeks to capture a more holistic physiological profile, acknowledging that emotional responses involve complex interplay across various systems, though the practical availability and synchrony of these data streams can be challenging.
5. Recognizing that physiological responses can vary significantly between individuals, a goal for these predictive models is to account for this variability. Developing methods to tailor or personalize models based on an individual's unique physiological patterns in response to stimuli is seen as crucial for improving the reliability and applicability of these tools.
6. Machine learning algorithms are well-suited to identifying non-linear relationships within complex datasets, which might be overlooked by simpler statistical methods. Applying these techniques to physiological data could potentially reveal subtler, non-obvious patterns in how HRV or other cardiac signals correlate with SAD symptom expression, offering a potentially deeper, albeit more complex, view.
7. There is theoretical potential for these methods to be applied to longitudinal datasets – tracking heart rate patterns over extended periods – to identify subtle shifts or trajectories that might precede significant increases in anxiety severity. This could hypothetically contribute to earlier identification or intervention, although demonstrating this prospect effectively relies on robust, long-term data collection under varied real-world conditions.
8. The identification of individual-specific physiological markers through machine learning analysis could hypothetically inform more personalized approaches to mental health interventions. If particular cardiac response patterns are reliably linked to specific challenges, it raises the possibility of tailoring therapeutic strategies, though translating physiological prediction into specific clinical action plans is not straightforward.
9. The widespread availability and increasing sophistication of wearable technology facilitates the collection of the continuous heart rate data needed to train and potentially deploy these machine learning models. As individuals accumulate larger personal datasets, the potential exists for models to potentially become more refined in their predictions for that specific person, provided data quality is sufficient and context is understood.
10. As research progresses towards clinical application, significant ethical considerations come sharply into focus. Using passive physiological data to infer mental health status raises important questions about data privacy, informed consent for data usage, the potential for unintended biases within models, and the risks associated with misinterpretation of results in a sensitive domain like mental health. These issues require careful deliberation.
Physiological Markers of Social Anxiety New Research Links Heart Rate Variability to SAD Severity (2025 Study) - Anticipatory Heart Rate Changes Signal Social Anxiety 20 Minutes Before Events
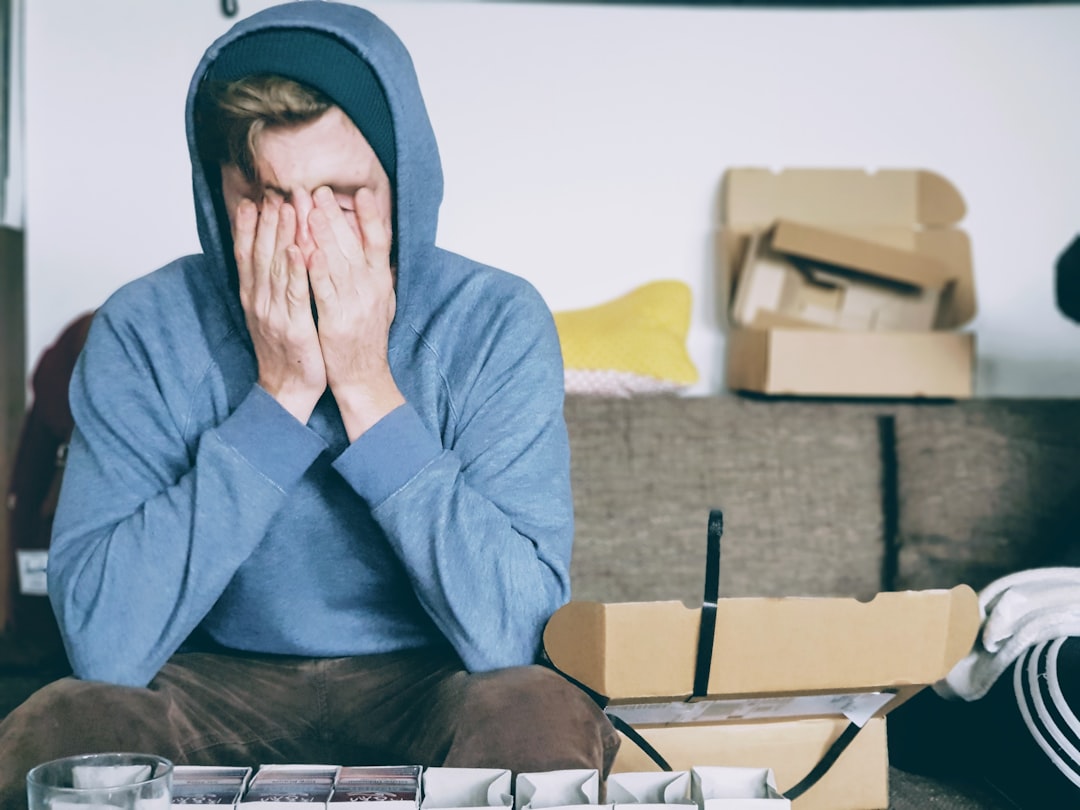
Emerging findings indicate that physiological responses tied to social anxiety disorder (SAD) can manifest even before a social interaction begins. Specifically, changes in heart rate anticipating social events, sometimes as early as 20 minutes beforehand, are being observed. This early physiological signal, encompassing shifts like increased heart rate and altered variability, appears tied to the anticipatory processing characteristic of the condition. Researchers propose that tracking these anticipatory heart rate patterns could offer a potential marker for assessing SAD severity and potentially monitoring the effectiveness of interventions specifically targeting anxiety prior to social situations. While the connection between anticipatory physiological changes and social anxiety is increasingly apparent, translating these observations into consistently reliable clinical tools remains an ongoing challenge, though they undeniably contribute to a deeper understanding of the disorder's physiological dimensions.
1. Observations suggest that physiological shifts, specifically in cardiac activity, can manifest a significant amount of time—potentially up to 20 minutes—prior to an impending social situation for individuals experiencing social anxiety.
2. This anticipatory phase appears marked by distinct physiological patterns, often involving an increase in heart rate or altered heart rate variability profiles, indicating the body's preparation response.
3. These physiological signals occurring *before* the event seem to reflect the process of anticipatory apprehension, a physical manifestation of the cognitive and emotional state leading up to social interaction.
4. The pattern of heart rate variability during this pre-event period is being explored as a potential physiological indicator that could correlate with the intensity of the individual's social anxiety at that moment.
5. Research points towards an activation of sympathetic system influences relative to parasympathetic control during this anticipation, preparing the individual for a perceived challenge before it even begins.
6. Tracking how cardiac physiological states transition from a resting baseline through this anticipatory stage and into the actual social exposure provides a more dynamic picture than solely focusing on event-locked responses.
7. There's a growing understanding that these anticipatory heart rate changes may occur somewhat automatically, potentially outside of the individual's conscious control or direct awareness.
8. Significant variation exists in the specific nature and magnitude of this anticipatory physiological response from one person to the next, suggesting the need for highly individualized physiological monitoring and interpretation.
9. While promising, establishing a clear, reliable link between these early physiological "alerts" and the precise nature or severity of the social experience that follows is complex and still requires substantial work.
10. The potential utility lies in identifying these pre-event physiological signatures as targets or indicators for interventions aimed at managing anticipatory distress, though turning this observational data into effective, tailored strategies is a non-trivial engineering and clinical challenge.
Physiological Markers of Social Anxiety New Research Links Heart Rate Variability to SAD Severity (2025 Study) - Remote Heart Monitoring Shows Promise in Early SAD Detection
Wearable technologies are emerging as potentially valuable tools for detecting signs that might suggest the early presence of Social Anxiety Disorder. These devices allow for the ongoing collection of physiological data, such as heart rate and its variability, offering a stream of information that researchers are exploring for links to social anxiety symptoms. The concept is that tracking these subtle bodily signals remotely could provide insights into an individual's state outside of traditional settings, potentially enabling quicker recognition of risk or symptom shifts. However, translating this continuous stream of complex physiological data into reliable, clinically meaningful indicators, especially considering how much individual variability exists and the potential for heightened self-focus associated with SAD itself, presents substantial hurdles. Nevertheless, incorporating remote physiological monitoring into mental health evaluations could theoretically support more personalized and proactive approaches to addressing social anxiety challenges.
Exploring physiological markers remotely, particularly via widely available wearable devices capable of tracking heart rate variability (HRV), is emerging as a research focus for potentially identifying early indicators of conditions like social anxiety disorder. The underlying hypothesis suggests that shifts in autonomic nervous system regulation, reflected in HRV patterns, might manifest subtly before overt symptoms of SAD become prominent or significantly impact daily life.
The appeal of this approach lies in the promise of continuous, non-intrusive data collection outside of controlled environments. Theoretically, observing an individual's cardiac rhythms over time in various real-world contexts could capture physiological responses tied to social or evaluative situations that might be missed in a clinic. Early work points towards patterns, sometimes involving decreases or altered regulation in HRV, that researchers are investigating as potential harbingers of increased anxiety vulnerability.
However, translating this potential into reliable tools for *early detection* presents substantial challenges. What constitutes an 'early' physiological signal distinct from normal human variability, individual baseline fluctuations, or responses to non-anxiety-related stressors is far from settled. Defining predictive thresholds and validating them against actual clinical trajectories over time requires extensive, complex longitudinal studies. Furthermore, relying solely on passive physiological data risks misinterpreting signals without behavioral or contextual information, which wearable data often lacks.
While the capacity of technology to collect such data is rapidly advancing, the algorithms and clinical frameworks needed to interpret it accurately for the specific goal of early mental health detection are still under development. The vision is that such monitoring *could* eventually serve as a prompt for further assessment or intervention, but it clearly does not offer a standalone diagnostic solution at this stage. It remains an active area of inquiry, navigating both the technical complexities of data analysis and the clinical nuances of applying physiological insights to predict or preempt complex mental health conditions.
More Posts from psychprofile.io: