AI-Powered Psychological Profiling - Gain Deep Insights into Personalities and Behaviors. (Get started for free)
The Interquartile Range A Robust Measure of Variability in Psychological Data Analysis
The Interquartile Range A Robust Measure of Variability in Psychological Data Analysis - Understanding the Interquartile Range in Psychological Data
The interquartile range (IQR) offers a valuable tool for comprehending the spread of psychological data. It represents the difference between the third quartile (Q3) and the first quartile (Q1), essentially capturing the middle 50% of the data. Unlike the full range, which can be heavily skewed by outliers, the IQR provides a more reliable representation of variability, making it particularly useful in psychological research where scores often deviate from a normal distribution.
Furthermore, the IQR facilitates outlier identification. Any data point falling beyond a specific range defined by Q1 and Q3, adjusted by 1.5 times the IQR, can be considered an outlier. This helps to ensure the integrity of the analysis by identifying potentially erroneous or extreme data points.
Box plots, a graphical representation of data, vividly illustrate the IQR as the box itself. "Whiskers" extend outwards from the box to the minimum and maximum non-outlier values. By understanding the IQR and its implications, researchers can gain deeper insights into the variability of responses in psychological studies, ultimately leading to more accurate and robust interpretations.
The interquartile range (IQR) is a valuable tool for understanding the spread of data in psychological research, particularly because it is less influenced by extreme values or outliers. It calculates the difference between the 75th percentile and the 25th percentile, offering a clear picture of the middle spread of scores without being distorted by extreme values. This is crucial in psychology, where data often exhibits skewed distributions due to the complexity of human behavior. For instance, when analyzing test scores or survey responses, the IQR can highlight the distribution of participant experiences that the mean alone might miss. It helps researchers delve into potential differences in behaviors or traits among demographics, providing a deeper understanding of the data than standard deviations can achieve. The IQR's ability to identify these nuances is particularly important in psychological research, where data often doesn't conform to a normal distribution.
Further, the IQR can be used to test assumptions about normality in data distributions. Data sets with wider IQRs might indicate more variability and complexity in the psychological constructs being studied. This highlights the growing understanding that variability is crucial in comprehending human behavior and mental processes. By moving away from solely relying on means, the increasing adoption of the IQR reflects a shift towards more robust statistical practices in psychological research. This shift fosters a better understanding of the diversity within data, allowing for more realistic and applicable findings that resonate with both academic and public audiences.
The Interquartile Range A Robust Measure of Variability in Psychological Data Analysis - Calculating IQR Step-by-Step for Mental Health Surveys
Calculating the interquartile range (IQR) is a crucial step in analyzing mental health survey data. It helps researchers understand the variability within the data, especially when dealing with potentially skewed distributions or outliers. To calculate the IQR, first, you must arrange the data in ascending order. Then, identify the first quartile (Q1), which represents the 25th percentile, and the third quartile (Q3), representing the 75th percentile. The IQR is simply the difference between Q3 and Q1: IQR = Q3 - Q1. This calculation essentially encapsulates the spread of the middle 50% of the data, offering a more reliable measure of variability than the full range which can be significantly influenced by extreme values.
Identifying outliers is also essential. Outliers are data points that fall beyond a certain range, which can be calculated using the IQR. Specifically, any data point exceeding 1.5 times the IQR above Q3 or below Q1 is considered an outlier. Outlier identification helps maintain the integrity of the data analysis by highlighting potentially inaccurate or extreme scores that might skew the results.
Understanding and calculating the IQR is a crucial step in analyzing mental health surveys as it allows for a deeper understanding of the data's variability, helping researchers to identify potential issues and arrive at more accurate conclusions.
The IQR shines when analyzing mental health surveys because many psychological constructs – like stress or anxiety – don't follow a predictable, "normal" distribution. This makes traditional measures like standard deviation less dependable in these cases.
The simplicity of calculating the IQR is surprising. It's a straightforward three-step process: find the first quartile (Q1), third quartile (Q3), and calculate the difference. Even researchers without advanced statistical knowledge can easily grasp and apply this method.
Beyond identifying outliers, the IQR helps highlight disparities in responses across various demographic groups, possibly uncovering issues of inequality or variance in mental health symptoms.
Often, mental health surveys collect vast datasets where traditional statistical measures can be misleading. The IQR accurately captures variability in such scenarios, evident in discrepancies between self-reported outcomes and clinical assessments.
The IQR's effectiveness in psychological research is aided by the ease of creating box plots, which visually represent the IQR and help communicate findings to both academic and lay audiences.
Researchers have observed that the IQR can detect shifts in population mental health, particularly during events like pandemics or economic crises, where rapid changes in psychological well-being may occur.
A larger IQR in survey responses could suggest a more diverse range of experiences among participants, highlighting the intricate nature of emotional and psychological responses rather than a simple, uniform explanation.
The IQR goes beyond being just a descriptive statistic; it informs inferential analyses, like testing for effects in experimental designs, guiding researchers in understanding how different treatments might produce varying results among participants.
Studies suggest that increased reliance on the IQR in research publications promotes greater transparency, encouraging researchers to articulate their findings beyond average trends and fostering a culture of thorough data exploration.
In longitudinal studies of mental health, tracking changes in the IQR over time can provide insights into evolving collective psychological trends, reflecting shifts in societal attitudes or the effectiveness of interventions.
The Interquartile Range A Robust Measure of Variability in Psychological Data Analysis - Advantages of IQR over Standard Deviation in Behavioral Studies
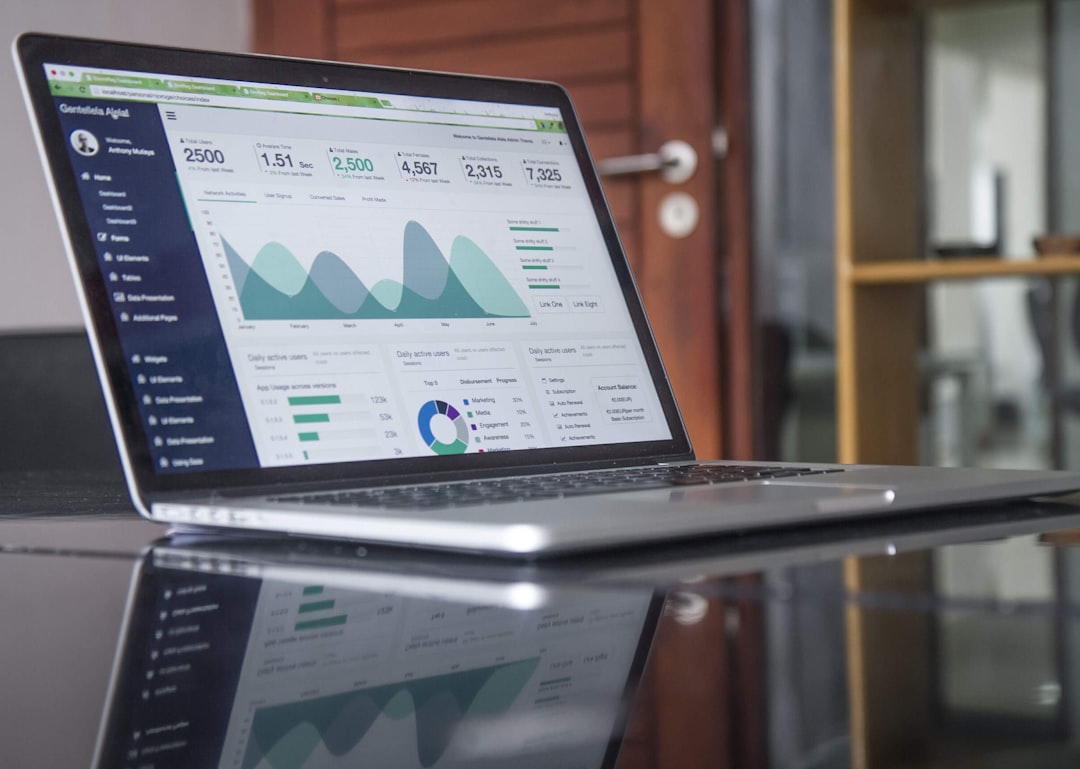
The interquartile range (IQR) offers several advantages over standard deviation in behavioral studies, especially when dealing with datasets that may contain outliers or exhibit skewed distributions. The IQR focuses on the central 50% of the data, meaning it is not influenced by extreme values that can distort the standard deviation. This makes the IQR a more reliable measure of variability in psychological research, where human behavior often leads to diverse and complex data. When dealing with data that doesn't conform to a normal distribution, the IQR can provide a more accurate picture of the data's spread. Additionally, the IQR allows researchers to easily identify outliers, which helps ensure the integrity of their analyses by highlighting potentially inaccurate or extreme scores that could skew results.
The IQR is often considered a more robust measure of variability than standard deviation, especially when analyzing psychological data, where outliers are common. This is because the IQR focuses on the middle 50% of the data, effectively minimizing the influence of extreme outliers. In contrast, standard deviation considers all data points, making it susceptible to distortion by outliers.
This robustness makes the IQR particularly valuable in situations where psychological data deviates significantly from a normal distribution. For instance, when studying anxiety levels, the distribution of scores may be heavily skewed, and the standard deviation may not accurately reflect the true spread of the data. The IQR provides a more reliable picture in such cases.
Beyond its statistical benefits, the IQR also offers practical advantages for researchers. Firstly, it is relatively straightforward to interpret. Researchers can readily understand the range of the middle 50% of the data, which facilitates communication with non-experts.
Secondly, the IQR provides a clear framework for identifying outliers. This helps researchers to maintain the integrity of their analyses by highlighting potential errors or extreme values that could distort the results.
Finally, the IQR complements median analysis, offering a powerful combination for exploring data in psychological research. This approach helps to avoid being swayed by extreme values, which can significantly affect both mean and standard deviation.
Using the IQR, researchers can explore differences in variability across demographics, potentially uncovering valuable insights that standard deviation might miss. This makes the IQR particularly useful in multi-group studies where comparisons are essential.
The dynamic nature of the IQR allows it to capture changes in psychological trends over time. This makes it a useful tool in studies assessing the effects of interventions, societal changes, or other factors on mental health.
Another advantage of the IQR is its simplicity and scalability. It is easy to calculate, even for large datasets, making it suitable for analyzing extensive surveys and other psychological research involving a significant number of participants.
Furthermore, the IQR’s direct relationship with box plots enhances its visualization capabilities. This facilitates easy understanding of data distributions, outliers, and overall data spread for researchers and stakeholders alike.
Ultimately, adopting the IQR encourages researchers to adopt more comprehensive exploratory data analysis practices. This means looking beyond conventional metrics and delving deeper into the intricacies of data variability and underlying patterns, ultimately leading to more robust and insightful conclusions.
Applications of IQR in Analyzing Mood Disorder Data
Analyzing mood disorder data is often complex due to the diverse range of symptoms and individual experiences. The interquartile range (IQR) provides a robust measure of variability, offering valuable insights into the spread of data, especially in cases where traditional measures like standard deviation are less reliable. The IQR captures the middle 50% of data, focusing on the differences between the first quartile (Q1) and the third quartile (Q3). This allows researchers to understand how closely grouped or dispersed the responses are, providing crucial information about the variability in mood disorder symptoms.
For instance, by analyzing the IQR of scores on a depression scale, researchers can determine if the middle 50% of participants experience a relatively narrow range of depression symptoms or if there's a wider distribution of symptom severity. This information can help inform clinical decision-making and treatment strategies.
Furthermore, the IQR can be particularly useful in longitudinal studies, where researchers track changes in mood disorder symptoms over time. The IQR can help identify if there are shifts in symptom variability, potentially revealing the effectiveness of treatments or the impact of external stressors on a population’s overall mental health.
By adopting the IQR as a tool for analyzing mood disorder data, researchers can gain a more accurate and nuanced understanding of the complexities of these conditions. This, in turn, can lead to more effective interventions and support for individuals struggling with mood disorders.
The interquartile range (IQR) stands out as a particularly valuable tool in analyzing data related to mood disorders. This is because the symptoms associated with these disorders often exhibit considerable variability. The IQR's ability to capture this variability is essential, as it reveals subtle differences in symptom severity that might go unnoticed using measures like means or standard deviations. This nuanced understanding of variability is crucial for uncovering the complex interplay of factors that contribute to mood disorders.
For instance, analyzing mood disorder data through the IQR can highlight disparities in treatment responses among different demographic or socioeconomic groups. This type of analysis could guide the development of more personalized approaches to mood disorder interventions, tailored to the specific needs of individual patients. The IQR is also a valuable tool for exploring the complex relationships between mood disorders and various factors, including genetics and environmental influences. It can reveal non-linear relationships, suggesting that the impact of these factors on mood regulation can vary significantly among individuals.
Further, the IQR's ability to maintain robustness in skewed distributions is especially relevant in longitudinal studies of mood disorders. These disorders frequently fluctuate over time, and the IQR can effectively track these changes and reveal trends that might be obscured by traditional metrics. This is important for understanding the long-term impact of treatments and for developing more effective strategies for managing mood fluctuations over time.
Furthermore, the IQR can be effectively paired with visual tools such as box plots. This combination provides a clear and intuitive way to communicate complex findings about mood disorders to non-technical audiences. This enhanced accessibility is essential for increasing public understanding of mental health issues and promoting more informed discussions about mental well-being.
The IQR can also play a vital role in the analysis of mood disorder data during crises. During events like natural disasters or social upheaval, the IQR can reveal shifts in population mental health by identifying changes in response variances that are directly linked to situational stressors. This data can be used to identify individuals in need of support and to develop targeted interventions that address the unique challenges of these crisis situations.
The IQR's robustness also allows for a more nuanced discussion of the effectiveness of various therapeutic modalities. Beyond just focusing on average clinical outcomes, the IQR allows researchers to explore variability in treatment responses and identify subgroups of patients who may benefit from different approaches. It can also help to identify unexpected outliers - individuals whose experiences differ drastically from the norm. These outliers could hold valuable insights into unique, yet underexplored, psychological profiles.
Furthermore, using the IQR in collaborations between multidisciplinary teams—such as psychiatrists, social workers, and data scientists—can provide a more holistic understanding of mood disorders. This allows for the incorporation of both clinical and contextual factors, leading to more comprehensive and effective interventions.
Finally, advances in data collection methods, such as smartphone applications for real-time mood tracking, are increasingly utilizing the IQR. These applications analyze daily fluctuations in mood and offer insights that can lead to real-time interventions for individuals in crisis. By harnessing the power of the IQR, researchers and clinicians can gain a more comprehensive and nuanced understanding of mood disorders, ultimately leading to more effective interventions and improved care for individuals facing these challenges.
The Interquartile Range A Robust Measure of Variability in Psychological Data Analysis - Interpreting IQR Results in Personality Assessment Research
Interpreting the interquartile range (IQR) in personality assessment research offers a valuable lens for understanding the spread of data, especially when traditional methods fall short. Unlike the standard deviation, which can be heavily influenced by outliers, the IQR focuses on the middle 50% of data, providing a more reliable picture of the central tendencies of participant responses. This is particularly useful in personality assessments, where data often exhibits skewed distributions due to the complex nature of human behavior.
By analyzing the IQR, researchers can pinpoint potential differences in personality traits across diverse demographics, revealing patterns that might be missed by simply relying on averages. Moreover, the IQR can be employed in longitudinal studies to track changes in personality traits over time, providing a dynamic understanding of individual and collective psychological transformations.
Essentially, integrating the IQR into personality assessment research facilitates a more robust and comprehensive exploration of the complexities of human personality. It offers a more nuanced understanding of data variability and trends, potentially leading to more accurate interpretations and insights.
The interquartile range (IQR) presents a valuable tool for analyzing psychological data, especially when traditional measures like the mean and standard deviation may not accurately reflect the data's complexities. The IQR captures the spread of the middle 50% of data, making it less susceptible to outliers, which are prevalent in psychological research. This robustness is crucial for accurately interpreting data in areas such as mood disorders, anxiety levels, and personality traits.
The IQR's versatility extends to analyzing shifts in population mental health, particularly during periods of crisis. It allows for the detection of symptom variability among individuals with mood disorders, leading to more tailored treatment approaches. Moreover, the IQR's straightforwardness promotes clearer communication of findings, making it easier for non-experts to understand the implications of research.
The IQR's value is amplified in longitudinal studies, where it reveals changes in symptom variability over time, providing insight into the effectiveness of interventions or shifts in societal conditions. This method can even identify disparities in treatment responses across various demographic groups, encouraging more personalized therapeutic approaches.
The IQR's visual representation through box plots enhances its communication capabilities, conveying complex findings to diverse audiences. Furthermore, the growing use of real-time data collection platforms for monitoring mood fluctuations demonstrates the IQR's adaptability and importance in contemporary psychological research, particularly in crisis management.
The Interquartile Range A Robust Measure of Variability in Psychological Data Analysis - Limitations and Considerations When Using IQR in Psychology
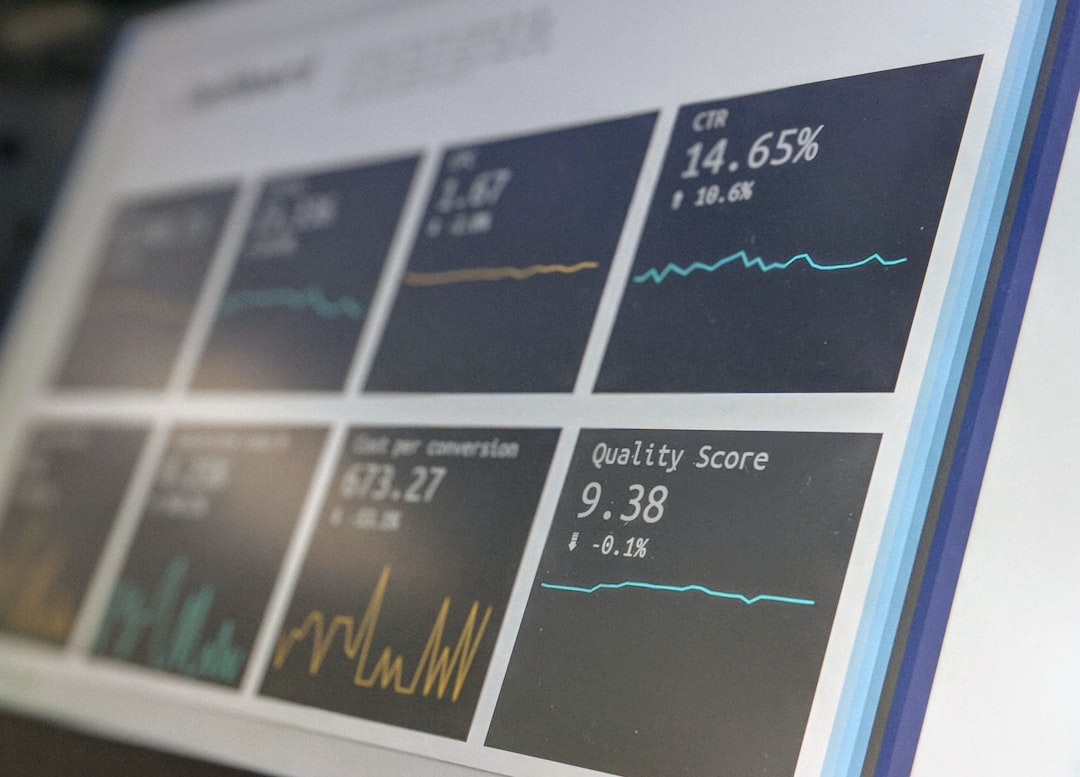
While the IQR offers a valuable tool for understanding data spread in psychology, it’s essential to be aware of its limitations. A significant drawback is its disregard for the data tails, potentially overlooking important nuances in psychological phenomena. This can lead to oversimplified conclusions about complex behaviors or traits, especially when the IQR is small.
Furthermore, outlier identification, a crucial aspect of IQR analysis, relies on subjective interpretation. Depending on the context, the definition of an outlier might vary, potentially influencing the analysis. Additionally, calculating the IQR can be computationally intensive, particularly with large datasets, especially if using software not optimized for handling such volumes.
It’s important to remember that the IQR doesn't account for variability within the quartiles themselves. This means that important insights about the distribution of responses within the middle 50% of the data may be missed. The IQR also overlooks information outside the first and third quartiles, potentially obscuring a complete understanding of psychological trends.
While the IQR excels with skewed distributions, extreme skewness might still introduce bias. This calls for careful analysis of the distribution shape to avoid misleading conclusions. Notably, the IQR’s sensitivity to the methodology employed for quartile calculation can also affect the analysis. Different methods, like inclusive vs. exclusive methods, can produce different quartile values, leading to variations in the IQR.
The ease of using the IQR can tempt researchers to over-rely on it, potentially neglecting other valuable statistical tools that could provide complementary insights into psychological datasets. It is critical to weigh the benefits of the IQR against its limitations to ensure accurate and comprehensive interpretations of psychological data.
AI-Powered Psychological Profiling - Gain Deep Insights into Personalities and Behaviors. (Get started for free)
More Posts from psychprofile.io: