The Role of Social Support Networks in Suicide Prevention A Data-Driven Analysis
The Role of Social Support Networks in Suicide Prevention A Data-Driven Analysis - The Correlation Between Social Media Usage and Suicide Prevention Outcomes 2024
The relationship between social media use and suicide prevention outcomes in 2024 presents a complex picture, especially within adolescent populations. A notable portion of teenagers who spend a considerable amount of time on social media experience poorer mental health and report higher rates of suicidal ideation compared to those with lower usage. This trend suggests that while social media can offer avenues for connection and support, it also harbors inherent dangers. These include online bullying, social comparisons that can lead to feelings of inadequacy, and exposure to detrimental content, which can contribute to heightened suicide risk. Furthermore, the recent introduction of legislation aiming to curtail social media use in certain areas underscores the growing concern about its impact on youth mental well-being, reflecting a growing societal acknowledgment of the issue. Effectively navigating the potential benefits and risks of social media remains crucial in developing comprehensive suicide prevention strategies.
Observational data suggests a potential link between heightened social media engagement and adverse mental health outcomes, specifically concerning suicide risk, particularly among adolescents. For instance, a substantial portion of teenagers who report heavy social media use also indicate poorer mental health compared to their less-engaged peers. This group also showed a higher incidence of suicidal ideation and self-harm, raising concerns about the platform's impact. Furthermore, a strong correlation exists between extensive social media consumption and issues like negative body image.
However, the relationship remains intricate and not entirely clear-cut. While some research points towards an association between frequent social media use and increased suicide risk, other studies yield contrasting results. This complexity highlights the need for careful interpretation and further investigation.
Contributing factors to this complex relationship seem to include phenomena like cyberbullying, exposure to potentially harmful online challenges, and the constant pressure of social comparison prevalent on many platforms. These elements can exacerbate pre-existing vulnerabilities in susceptible individuals, potentially leading to amplified suicidal thoughts and behaviors.
Intriguingly, the concept of perceived social support emerges as a potentially crucial mediating element within this interaction. This suggests that the quality of online interactions and the sense of belonging derived from them might play a vital role in shaping the influence of social media on an individual's mental well-being. Leveraging platforms for disseminating suicide prevention information, especially during periods of heightened risk, may hold significant promise. This approach underscores the potential for social media to play a role in improving access to resources and promoting proactive support.
The multifaceted nature of this connection between social media and suicide risk calls for a cautious and nuanced perspective. While some individuals clearly benefit from online social support networks, others may find themselves exposed to detrimental impacts, highlighting the need for context-sensitive, potentially personalized, intervention strategies that can mitigate harm and enhance positive outcomes. This emphasizes the ongoing challenge for researchers and policymakers to unravel the interplay of social media, mental health, and suicidal behaviors in order to optimize the use of these pervasive platforms for the benefit of users.
The Role of Social Support Networks in Suicide Prevention A Data-Driven Analysis - Community Response Teams Reducing Crisis Situations Through Digital Intervention
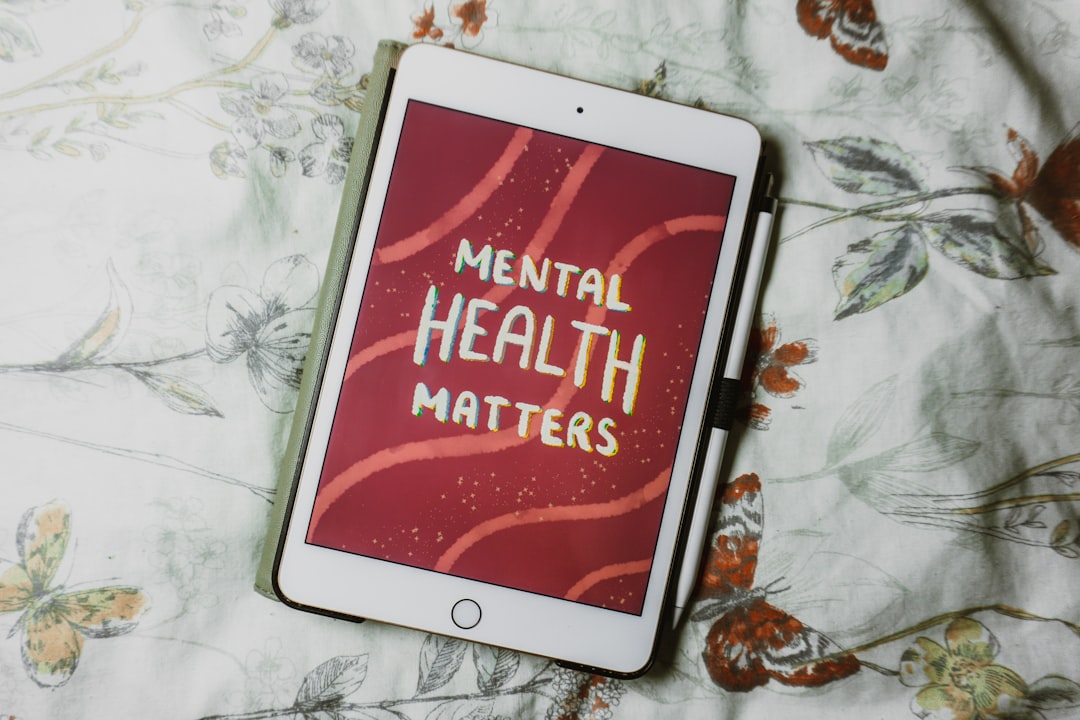
Community response teams are increasingly using digital tools to intervene in crisis situations, particularly those involving suicidal thoughts or behaviors. A core aspect of their work involves primary prevention—efforts aimed at preventing the onset of suicidal crises through education and outreach. By utilizing online platforms, these teams can create safe spaces for individuals to discuss suicide-related issues, thereby reducing the risk of potentially harmful events like suicide clusters.
Furthermore, community response teams are recognizing the critical importance of social support networks. They actively promote connection and understanding as a vital buffer against suicide risk factors. The integration of digital tools into the fabric of community crisis response allows for more accessible and timely support, ensuring individuals experiencing a crisis can easily access resources and assistance. As the nature of human interaction continues to evolve alongside digital technologies, the role of these teams and their capacity to adapt through digital channels becomes even more critical in mitigating and preventing future crises. While there are still many unknowns in this field, the potential for digital platforms to foster connectedness and enhance community-based support systems is an area that deserves continued research and development.
Community response teams, often focused on broader suicide prevention efforts, are increasingly incorporating digital tools into their strategies. These teams aim to not only support individuals dealing with suicidal thoughts or attempts but also work towards preventing future crises through education and early intervention referrals.
The idea is that social media, with its ability to connect individuals and facilitate discussions, can become a platform to address suicide-related issues safely, particularly in situations involving clusters of suicide events. While research in this area is still nascent, with only a handful of studies examining digital interventions for suicide prevention in adults, the interest in this approach is growing.
We know that strong social connections and community ties act as buffers against suicide risk, effectively mitigating the impact of contributing factors. This underscores the importance of suicide prevention programs that foster social connectedness and support. Peer support models, for example, have been shown to be particularly beneficial, especially for those struggling with mental health issues or dealing with the loss of someone to suicide.
Organizations like the Centers for Disease Control and Prevention are emphasizing the need for coordinated community responses to address suicide clusters, advocating for structured support networks. It's becoming increasingly clear that crisis response teams need to focus on preventive measures and postvention activities to minimize the likelihood of future suicides. Early identification of individuals at higher risk and providing them with prompt access to treatment and crisis intervention services is key to preventing recurrence of suicidal behaviors.
It's still important to note that digital interventions are not a panacea and raise questions of data privacy, algorithmic bias, and access equity. Further investigation is needed to determine how to implement digital interventions effectively and safely. Nevertheless, the potential for crisis intervention via digital platforms warrants closer examination as it holds the promise of improving reach and potentially leading to faster, more effective interventions for those in crisis.
The Role of Social Support Networks in Suicide Prevention A Data-Driven Analysis - Data Analytics Supporting Early Warning Systems in Mental Health Networks
Data analytics is emerging as a vital tool in the development of early warning systems (EWS) within mental health networks, particularly given the increasing prevalence of adolescent mental health challenges like depression and anxiety. Traditional approaches to identifying early warning signs often rely on subjective methods, which haven't proven sufficiently robust. This has spurred the development of advanced predictive models that utilize large datasets, including a focus on gender-specific social media usage patterns, to pinpoint individuals at higher risk for mental health crises. Novel techniques, such as the integration of convolutional neural networks (CNN) and long short-term memory (LSTM) models, are being explored to detect these early signs, highlighting the potential of big data for automating and improving mental health care services. While this potential is promising, there are important questions surrounding the accuracy, privacy implications, and ethical considerations of integrating such analytics into mental health practices. These issues demand careful examination as the field continues to advance.
The field of mental health is increasingly leveraging data analytics to enhance early warning systems and improve outcomes. Machine learning is showing potential in recognizing patterns associated with suicidal behavior, enabling us to anticipate potential crises based on behavioral cues, rather than solely relying on individuals' self-reports. This shift towards a more proactive approach is being driven by the integration of data from various sources, including medical records, social media interactions, and even data from wearable devices, offering a more comprehensive understanding of an individual's state.
Using geospatial analysis, researchers are identifying communities with a higher incidence of suicide-related issues, leading to the possibility of more focused intervention efforts. Understanding the structure and influence of social support networks through network analysis provides insights into how connections and relationships impact individuals' mental health. Examining sentiment on social media through tools like sentiment analysis can offer valuable information about how the public perceives mental health challenges, allowing us to craft more effective community response strategies.
While data-driven evaluations of digital outreach methods indicate that personalized online interventions can be effective in engaging at-risk individuals, there are also ethical and practical concerns about the use of algorithms in mental health. The transparency and potential biases of these algorithms need to be carefully considered. If not, we risk creating interventions that unintentionally harm certain groups.
Another exciting area is surge detection, which leverages algorithms to monitor increases in crisis indicators such as online searches related to suicide or spikes in emergency room visits. Such monitoring allows a swift response by community and health systems during times of potential crisis. It's vital that these systems incorporate feedback loops to refine the predictive models and ensure interventions are continually improving over time.
The integration of data analytics into mental health care requires diverse expertise, demanding collaboration between data scientists, mental health professionals, and community organizations. This interdisciplinary approach is essential to effectively manage the complexities of suicide risk and develop comprehensive strategies to support at-risk individuals. While the field is still developing, it's clear that a more data-driven approach holds promise for improving mental health outcomes.
The Role of Social Support Networks in Suicide Prevention A Data-Driven Analysis - Geographic Distribution of Mental Health Support Groups and Impact Metrics
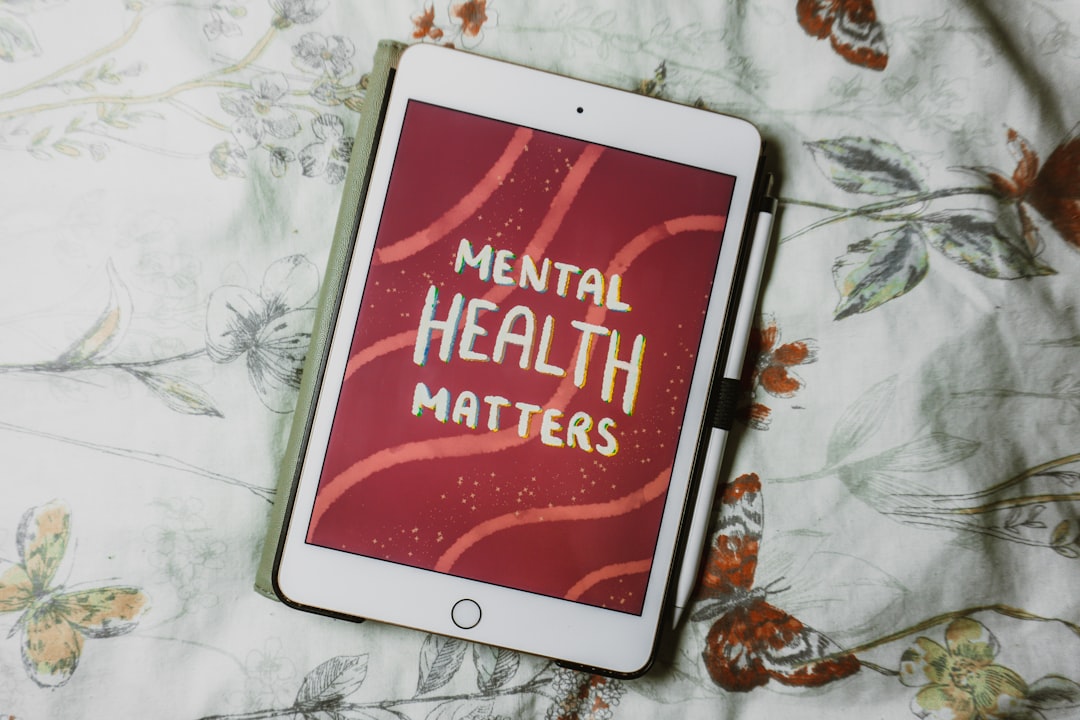
The availability of mental health support groups varies considerably across different geographical locations, highlighting a crucial aspect of suicide prevention. Some areas may boast a wealth of resources and readily accessible support networks, while others, especially those in rural settings or underserved urban communities, experience a significant shortage of mental health services. Recognizing these geographical disparities is key to implementing targeted strategies that address the specific needs of diverse populations. Moreover, evaluating the effectiveness of support groups through impact metrics is essential. This involves closely tracking whether these networks demonstrably contribute to lower suicide rates and improved mental health outcomes within the communities they serve. By analyzing the geographical distribution of these resources and their demonstrated impact, stakeholders can refine their approach to expand the reach and improve the efficacy of mental health support initiatives. This ensures that individuals in all regions, regardless of location or background, have equitable access to support and are less susceptible to suicide risk due to geographical barriers. There is a need to continually evaluate the effectiveness of interventions in these regions and monitor if interventions are creating further disparities within mental health service availability.
The distribution of mental health support groups across different regions isn't uniform. Urban areas generally have a higher density of these resources compared to rural communities, which creates a disparity in access for individuals facing mental health challenges. This geographic imbalance likely plays a role in observed differences in suicide rates between these areas, although more research is needed to understand the specific impact.
Cultural factors and how people perceive mental health within a given community have a large influence on the efficacy of support groups. Norms around discussing mental health, and the degree of social stigma surrounding help-seeking behavior, likely vary across regions, affecting participation rates. For instance, we might find that in some regions where open discussions of mental health aren't as common, participation rates in support groups are lower, potentially hindering their usefulness.
Population density seems to play a role as well. Smaller communities may have higher rates of suicide, and this could be connected to the nature of social networks in smaller areas. Anonymity is potentially a barrier to seeking support in smaller communities where everyone might know everyone else, potentially causing people to avoid support groups. This emphasizes the need for support networks to be mindful of creating a safe and anonymous space for individuals.
While traditional in-person support groups have a long history and provide a face-to-face human connection, digital options have expanded access and potentially reduced stigma for some. Preliminary data suggest online support groups may be particularly attractive to adolescents who may feel more comfortable communicating in a digital setting. However, we need to investigate whether these platforms offer the same quality of relationship and support that traditional in-person groups offer for the long-term. It seems likely that the best approach might be to offer a variety of support options to address different individuals’ preferences.
Another observation is that socioeconomic factors and the availability of support groups seem related. Areas with lower average incomes may have fewer support group resources and higher rates of suicide, highlighting a clear need for targeted interventions to bridge this gap. Possibly, initiatives that connect mental health professionals with underserved areas could increase access to resources and help close this gap.
Further complicating this, language differences can hinder access to support groups, especially in areas with a high degree of cultural diversity. This reinforces the importance of tailoring intervention efforts to the linguistic and cultural makeup of the population. Translation and cultural sensitivity training for support group leaders could be crucial for effectiveness.
Analyzing attendance data in support groups reveals that participation patterns fluctuate over time. We've observed an increase in participation during the colder months, suggesting possible links to seasonal affective disorder and holiday-related stress. This temporal variation highlights the importance of maintaining access to support consistently throughout the year, particularly during periods when individuals may be more vulnerable to mental health challenges.
Looking ahead, newer methods in digital analytics allow researchers to map interactions within online support networks. By mapping interaction patterns and engagement levels in online support groups, researchers may be able to identify groups or communities at higher risk before crisis levels escalate, allowing for early intervention. This potential ability to identify vulnerable groups early on is a promising development, although ethical and privacy concerns need to be carefully addressed before this area is widely adopted.
Research also suggests that support groups led by peers can have a more substantial impact than those led by professionals. The ability of peers to relate to participants and understand their experiences could be essential to creating trust and fostering a sense of belonging. This highlights a growing realization that support comes in many forms and that empathy and shared experience are important elements in fostering resilience.
Finally, support group involvement correlates with higher reported recovery rates compared to individuals who only rely on traditional therapeutic approaches. The social interaction and connection found in support groups seem to be a crucial part of mental health recovery, suggesting that the impact of social connections on overall mental well-being is an area that warrants further exploration. These connections provide a valuable element of resilience for individuals who might otherwise face mental health challenges in isolation.
The Role of Social Support Networks in Suicide Prevention A Data-Driven Analysis - Machine Learning Models Identifying At Risk Behavior Patterns in Support Networks
Machine learning models are showing promise in identifying concerning behavior patterns within social support networks, especially in relation to suicide prevention. By processing large datasets of online interactions, these models can identify subtle behavioral clues that might suggest an individual is facing a mental health crisis. This capability holds potential for developing targeted interventions, such as those for individuals recently discharged from mental health facilities or those exposed to higher-risk online environments. Nonetheless, utilizing these models raises concerns regarding data privacy, the potential for algorithmic biases, and ethical considerations. It is imperative that these models are deployed cautiously and continuously evaluated to ensure they are used responsibly and effectively. While the potential benefits are encouraging, the complexity of human behavior necessitates a careful and nuanced approach to fully leverage the potential of machine learning for supporting mental health initiatives and preventing crises.
Machine learning is increasingly being explored as a tool to improve the prediction of suicide risk, offering novel ways to identify individuals who might be at risk. Researchers have looked into how well these models perform in predicting suicide attempts, examining studies published in databases like PubMed, Scopus, and Web of Science.
One interesting area is the development of personalized machine learning models that can forecast suicide risk, particularly for those who've just been discharged from psychiatric care. This approach could be crucial in providing timely interventions during a critical period. There's also growing interest in leveraging machine learning to analyze social media data. The hope is that analyzing patterns over time can shed light on the environmental factors that influence suicidal thoughts and behaviors.
However, it's important to acknowledge that things like culture, gender, and personal information are significant factors that must be considered when using social networks for mental health assessments. A recent analysis revealed how machine learning techniques, particularly data mining methods, are commonly employed to extract features from social network data for suicide risk prediction.
It appears that combining data from multiple sources, a process called information fusion, can enhance the accuracy of machine learning models in predicting suicide risk. Recent studies have explored both the potential and the limitations of using machine learning for mental health applications.
Interestingly, roughly half of the studies focusing on machine learning for suicide risk explicitly used data mining methods to refine the performance of their models. The rise of artificial intelligence (AI) and machine learning has provided researchers with powerful tools for sifting through massive datasets, searching for patterns in suicidal behavior and aiming to inform prevention efforts. While promising, the field is still in its infancy and raises many important questions that need to be addressed. There is still much to learn about how these models function and their implications, especially when it comes to the potential for biases and how that could affect the effectiveness and fairness of intervention programs.
The Role of Social Support Networks in Suicide Prevention A Data-Driven Analysis - Network Analysis Methods for Measuring Social Connection Effectiveness
Network analysis offers a fresh perspective on understanding the effectiveness of social connections, particularly within the context of suicide prevention. By mapping the complex web of relationships within support networks, we can better grasp how social capital impacts mental health outcomes. This approach, often referred to as social network analysis (SNA), enables a deeper dive into the dynamics of these support systems, allowing us to pinpoint crucial individuals and relationships that serve as protective barriers against suicidal thoughts and actions. A combination of both quantitative data analysis and qualitative insights provides a richer understanding, guiding us towards more focused interventions tailored to the specific nature of each network.
Despite the promise of SNA, it's important to acknowledge the inherent subjectivity involved in selecting and interpreting the measures of social connections within a network. This subjectivity introduces a degree of complexity into the application of these methods, demanding careful thought and consideration in how we employ them for understanding social support. It's crucial to remain mindful of the limitations and potential biases introduced when trying to represent the multifaceted nature of human relationships with data-driven methods.
Social connection effectiveness in suicide prevention can be explored through network analysis methods, offering insights into the role of social support. Research suggests individuals embedded in strong social networks tend to exhibit greater resilience towards suicidal ideation, highlighting the protective effects of healthy relationships. Furthermore, communities characterized by high social engagement generally report lower suicide rates, suggesting a direct relationship between active social participation and mental health.
However, analyzing network interactions using algorithms isn't without limitations. While these tools can potentially identify individuals at risk by analyzing interaction patterns, facilitating timely intervention, social media's inherent duality presents a challenge. Network analysis has unveiled how seemingly supportive online environments can also harbor harmful interactions, potentially undermining the efficacy of virtual support networks.
Predictive modeling, while advancing, struggles to capture the nuances of intricate social relationships, emphasizing the complexities inherent in precisely measuring connection strength and effectiveness. This highlights the need for continual algorithm refinement. Network analysis can also illuminate "weak links" within social structures – individuals who might be prone to disconnecting from support systems during periods of crisis. This allows for targeted outreach strategies towards these potentially vulnerable individuals.
Interestingly, network analysis sometimes reveals paradoxical patterns. For instance, individuals with numerous acquaintances might paradoxically report feeling more isolated, underscoring that mere connection quantity isn't a reliable indicator of support effectiveness. Furthermore, defining supportive behaviors must account for cultural diversity, as societal norms and expectations surrounding support can differ considerably across communities.
Examination of online support groups through network analysis has revealed specific engagement patterns. Those individuals who actively participate in these groups frequently report higher satisfaction levels and enhanced mental health outcomes, reaffirming the value of engagement in these social structures. Moreover, longitudinal network analysis has shown that informal, peer-led support networks can sometimes foster greater resilience against suicidal thoughts compared to more traditional, professional interventions. This challenges established frameworks for providing support and indicates the evolving nature of social support.
In essence, network analysis provides a valuable lens through which to study the intricate dynamics of social connections and their impact on suicide prevention. It underscores the importance of healthy relationships while also revealing the complexities and potential pitfalls of digital interactions within support networks. While these methods are constantly developing, the potential for using network analysis to better understand social connection dynamics and inform targeted intervention strategies remains promising.
More Posts from psychprofile.io: