The Scientific Method in Psychology Analyzing Research Methodology Changes from 2020-2025
The Scientific Method in Psychology Analyzing Research Methodology Changes from 2020-2025 - Major Shift From Standard P Values To Bayesian Methods In Experimental Psychology Research
Between 2020 and 2025, experimental psychology saw a considerable evolution in its statistical practices, marking a departure from the entrenched dependence on traditional p-values. This transformation is a response to ongoing discussions and critiques regarding the interpretability and limitations of the standard null hypothesis testing framework. While frequentist methods, including p-values, have historically been the norm, Bayesian statistical approaches began to attract increasing interest and adoption during this period. These methods are often perceived as offering a more informative way to interpret evidence from data, and they appear particularly useful in studies where obtaining large sample sizes is challenging, such as in certain areas of experimental psychopathology research. The growing integration of Bayesian techniques signals a wider push within the field to enhance the robustness, clarity, and reliability of research findings, aligning with a broader movement towards refining the application of the scientific method in psychology.
Observing the landscape of experimental psychology research between 2020 and 2025 reveals a palpable movement away from a singular reliance on standard p-values and towards Bayesian methodologies. It's become increasingly common to encounter studies deploying Bayesian approaches for tasks like estimating parameters or directly testing hypotheses, offering an alternative perspective to the more familiar territory of confidence intervals and threshold-based p-values. Scrutinizing the published literature over this period confirms this trend, showing a steady uptick in articles featuring Bayesian analyses – a notable shift considering how deeply frequentist statistics have been embedded in the field for decades.
This growing adoption appears fueled by several factors. The limitations of relying solely on traditional null hypothesis testing and p-values have become starkly apparent; they don't quite answer the questions researchers intuitively want to ask, like "What is the probability of my theory being true given this data?" Bayesian methods, by allowing for the incorporation of prior knowledge and directly calculating the probability of hypotheses, offer a potentially more intuitive and informative framework. Furthermore, these methods can offer more stable conclusions particularly when working with smaller sample sizes, which is a recurring reality in many experimental psychology subfields, including aspects of psychopathology research. However, this transition isn't without its complexities. The degree of influence prior beliefs can have on the results raises questions about the subjective nature of the process and potential implications for the reproducibility of findings, points worth considering from an analytical perspective. Yet, advancements in accessible software have undeniably smoothed the path for researchers less steeped in statistical theory, making these powerful tools more practical to implement. Ultimately, this statistical evolution feels like part of a broader disciplinary conversation about what constitutes robust evidence and how we can interpret our data with greater transparency and nuance.
The Scientific Method in Psychology Analyzing Research Methodology Changes from 2020-2025 - How Open Science Framework Changed Data Sharing In Clinical Studies 2023
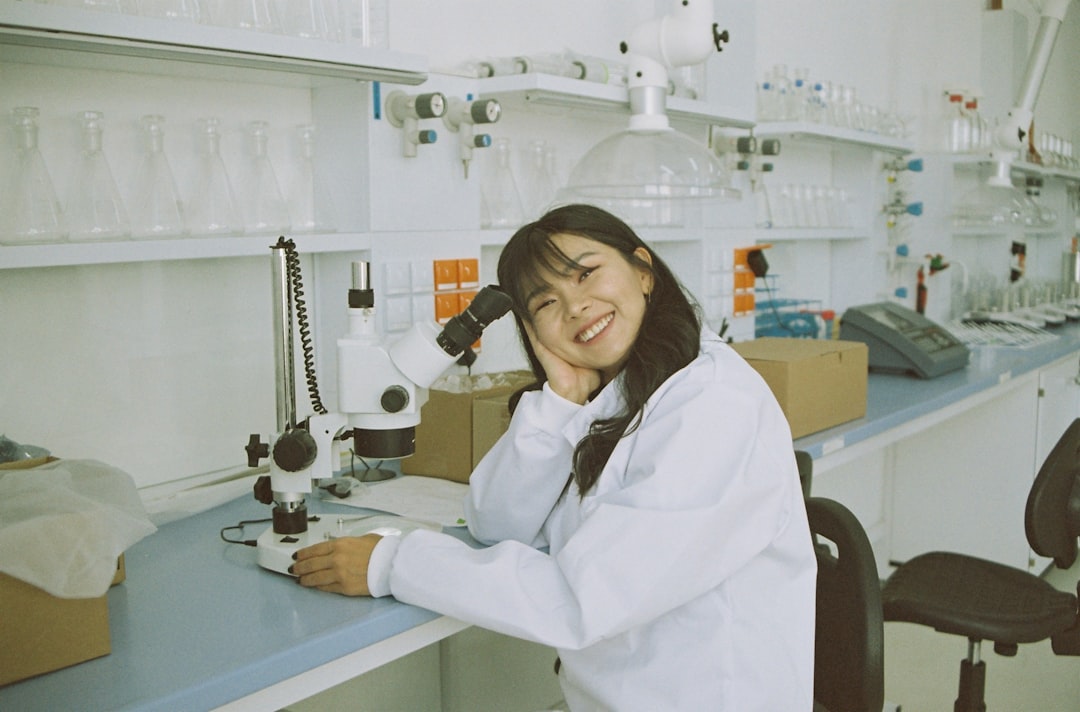
The Open Science Framework has certainly marked a significant evolution in how data generated within clinical studies, particularly those in psychology, is managed and disseminated. It has fundamentally altered the landscape by promoting practices designed to increase openness and facilitate collaborative efforts among the scientific community. Central to this shift has been the advocacy for making research materials more accessible. This includes encouraging the registration and public posting of study designs before data collection begins, outlining planned hypotheses and analytical approaches. Beyond this, the framework supports the availability of de-identified datasets and the accompanying code used for analysis. These actions are intended to enable closer examination, validation, and wider utilization of research findings, thereby aiming to strengthen the overall credibility and consistency of scientific outcomes. Nevertheless, pursuing such openness introduces complexities requiring careful consideration of ethical responsibilities, most notably navigating the balance between ensuring participant privacy and striving for maximum data transparency. While practical challenges inherent in reconciling these needs can arise, the general push towards more open data practices continues to influence how clinical research is carried out and shared.
The Open Science Framework, or OSF, has noticeably reshaped how data is managed and shared within clinical studies, particularly within psychological research over the 2020-2025 period. One observes a clear push towards making more elements of the research process publicly accessible. This includes, importantly, registering study protocols *before* data collection begins – detailing hypotheses, planned variables, and analysis strategies. Beyond the upfront planning, the platform has become a central repository for sharing preprints of findings, the actual materials used in studies, de-identified datasets once collected, and the analytic code applied. These steps collectively appear designed to inject greater transparency into the pipeline, making studies easier to inspect, replicate, and build upon, addressing persistent questions about the robustness of published results.
Efforts like the 2023 focus on open science seem to have accelerated this trend, encouraging a formal adoption of open practices across various research domains and funding bodies, which filters down into clinical psychological science. While the benefits of increased transparency are widely acknowledged – promoting trust and facilitating collaborative work – the practical implementation presents ongoing challenges. Grappling with the ethical considerations surrounding sensitive participant data in an open environment remains crucial. Developing and adhering to robust guidelines for de-identification and responsible data sharing are constant topics of discussion among researchers aiming to balance the imperative for openness with the need to protect privacy. Despite these complexities, the general trajectory suggests a growing recognition within the research community that moving towards more open data practices is not just a methodological shift, but a fundamental aspect of enhancing the collective credibility and progress of the scientific enterprise.
The Scientific Method in Psychology Analyzing Research Methodology Changes from 2020-2025 - Computer Vision Analysis In Behavioral Psychology Labs At Stanford
The integration of computer vision approaches within behavioral psychology research, particularly visible in labs like those at Stanford, marks a significant methodological evolution from 2020 to 2025. This technology offers a path toward automating the detailed analysis of complex behaviors and non-verbal signals, potentially generating quantitative data with a level of granularity previously unattainable through traditional human observation. The ability to capture and process subtle movements or expressions at scale holds promise for shedding light on nuanced psychological phenomena. However, translating raw visual data into psychologically meaningful variables requires careful consideration, presenting challenges in ensuring the validity and interpretability of the algorithmic outputs. This shift reflects a broader movement toward incorporating advanced computational tools into psychology, aiming to enhance the precision of behavioral analysis within the evolving landscape of research methodologies.
The application of computer vision technology within behavioral psychology laboratories, notably in settings like Stanford, is markedly reshaping how researchers approach the measurement and analysis of human conduct. This involves deploying algorithms to automatically analyze rich visual data – think video recordings of interactions, expressions, or movements. The appeal lies in the potential to move beyond often subjective or labor-intensive manual coding, extracting quantitative metrics from non-verbal cues with a precision previously difficult to achieve. This enables a more granular and perhaps more objective examination of subtle behavioral dynamics, which can be crucial for dissecting complex psychological phenomena. Efforts ongoing at places like Stanford's Vision and Learning Lab seem aimed squarely at developing the underlying capabilities to interpret visual information in ways meaningful for understanding human cognition and behavior.
The period spanning 2020 to 2025 has certainly seen an accelerated interest in integrating these visual analysis tools. Processing large volumes of video data that would be intractable for human coders becomes feasible, potentially uncovering patterns that manual methods might miss entirely. However, this technological shift isn't without its complexities. Implementing these systems effectively requires navigating challenges related to the computational resources needed and, significantly, understanding the potential biases inherent in the algorithms themselves, which are often trained on specific datasets and might not generalize well across diverse populations or contexts. Furthermore, the ethical landscape shifts considerably when automated systems are used to analyze sensitive behavioral data, raising crucial questions about data privacy, consent, and how findings derived from such analyses are interpreted and applied. Researchers are grappling with how to incorporate these powerful new tools while maintaining rigorous scientific standards and ethical responsibility.
The Scientific Method in Psychology Analyzing Research Methodology Changes from 2020-2025 - New Guidelines For Publishing Replicated Studies In APA Journals
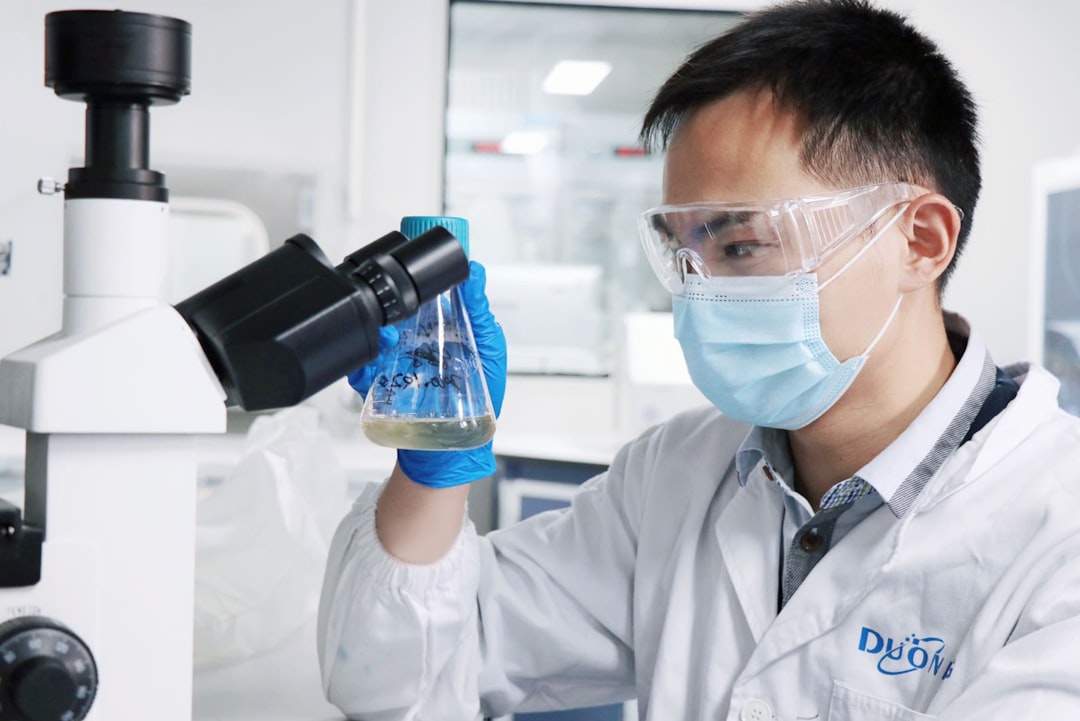
The American Psychological Association has put forward updated guidelines concerning the publication of replicated studies. This development represents an effort to strengthen transparency and methodological exactitude within psychological science. The revised standards specify what information authors should include when reporting on replications across various research structures, including studies involving single individuals or clinical trials, with the goal of fostering more complete and standardized reporting. These guidelines also highlight the importance of conducting methodologically sound research before disseminating new discoveries, connecting with broader movements in the field towards increased openness and the availability of research data. As the nature of academic publishing continues to shift, the APA's emphasis on replication and careful reporting points to the ongoing necessity of producing psychological research findings that possess credibility and can be consistently reproduced.
The recent shifts in the APA's guidance for publishing replicated studies highlight a clear push for increased openness and methodical rigor. The stated goal seems to be to directly address the ongoing challenges around reproducibility by mandating comprehensive reporting of methods and details on data availability. This, in theory, makes it far more practical for another researcher to pick up a published study and attempt to verify its findings. A notable aspect is the distinction drawn between simply running a study again with the same setup (direct replication) and trying to see if the underlying concept holds true with different methods or populations (conceptual replication). Both are seen as vital for confirming the robustness of a psychological effect.
Beyond just reporting results, the guidelines appear to encourage a more communicative approach within the research community. Authors are prompted to share not just whether a replication "worked," but also the practical hurdles and insights gained during the process. This feels like an effort to build a shared knowledge base on the intricacies of replicating specific phenomena. Importantly, the guidelines explicitly open the door for publishing replication attempts that find null results or conflict with the original findings, a necessary countermeasure to the historical bias favoring only positive outcomes, which tends to paint an overly rosy picture of the literature.
Interestingly, the APA has also put forward some criteria for assessing the "quality" of a replication study. Having a framework to evaluate the rigor and potential impact of a replication seems reasonable, although how consistently this is applied across different journals remains to be seen. This focus on replication hasn't been without discussion, prompting debates about the ethics of conducting and interpreting replication studies, particularly concerns that negative findings could be used to unfairly criticize original work without sufficient contextualization.
To mitigate potential biases in the replication process itself, there's a strong encouragement towards pre-registering replication study protocols, outlining hypotheses and planned analyses before data collection begins. This aligns with broader trends towards increased transparency and reducing researcher degrees of freedom. These updated guidelines also feel integrated with the availability of modern technological tools that facilitate easier data sharing and collaboration, which are essential for large-scale replication efforts. Furthermore, the guidance suggests that replication studies should go beyond simple repetition and consider exploring whether contextual factors might influence outcomes, acknowledging that psychological effects can be sensitive to study specifics or participant characteristics. Ultimately, this emphasis on replication appears to be part of a wider movement within psychology aiming to strengthen its scientific foundations and enhance the trustworthiness of its published findings.
The Scientific Method in Psychology Analyzing Research Methodology Changes from 2020-2025 - Machine Learning Integration In Research Methodology Classes
Machine learning approaches are increasingly finding their way into psychology research methodology, signaling a shift in how researchers analyze data and understand psychological phenomena. This integration often emphasizes prediction and pattern discovery using model-agnostic techniques, offering an alternative perspective to the traditional focus on statistical inference and hypothesis testing. The application of these methods is seen as potentially valuable for addressing longstanding challenges, including enhancing the precision of experimental outcomes and contributing to efforts aimed at improving replicability. While machine learning has demonstrated significant utility in specific areas, such as handling complex multivariate data or working with biological and genetic markers, its pervasive influence across all subfields of psychological inquiry has been less uniform. Effectively leveraging these computational tools requires a deep understanding of the methods themselves, highlighting the ongoing importance of rigorous training in their proper design and application within psychological contexts. The evolving landscape suggests that future research increasingly involves navigating how best to integrate these powerful techniques while maintaining foundational scientific principles.
Across psychology programs, integrating machine learning into research methodology coursework is noticeably reshaping how students approach data analysis. It feels like a significant pivot, moving beyond traditional statistical inference frameworks toward grappling with predictive modeling and deriving insights directly from often complex, large datasets. This isn't just about adding new tools; it's about fundamentally changing how researchers-in-training interact with information.
These advanced algorithms offer the capability to dissect vast data pools in ways previously unfeasible for human analysts alone, potentially uncovering subtle patterns and correlations that might spark entirely new lines of inquiry. The promise is that automating significant portions of the analysis pipeline using machine learning could minimize some sources of human error and inherent bias, aiming for outcomes that are, in theory, more robust.
Yet, introducing these powerful computational methods isn't straightforward. It immediately raises thorny questions about data privacy, especially with complex datasets, and highlights the crucial need to critically examine biases embedded within the training data used for models. How do we ensure fairness and representativeness when the core tools themselves can reflect societal inequalities?
Navigating this landscape requires a blending of perspectives. The push towards machine learning is naturally fostering a more interdisciplinary mindset, nudging psychology students to gain fluency in concepts from computer science and data science. This evolution addresses a burgeoning skill gap, demanding proficiency not just in statistical theory but also in programming and data wrangling techniques necessary to implement and manage these models.
This technological infusion is also opening doors to entirely new subfields, like the computational approaches seen in affective computing or neuroinformatics, expanding the traditional boundaries of psychological exploration. Imagine processing streams of real-time data from digital interactions or physiological sensors; machine learning makes this conceivable, offering snapshots of psychological states or behaviors as they unfold.
However, a significant hurdle remains the interpretability challenge. Many sophisticated machine learning models function much like a "black box," producing highly accurate predictions but making it remarkably difficult to understand *how* they arrived at their conclusions. This opacity can complicate the scientific process of explaining phenomena and communicating findings effectively to the wider community.
Working through these technical and conceptual complexities often encourages collaborative learning settings. Students are finding themselves needing to work in teams, pooling diverse skills and perspectives to effectively manage datasets, build models, and critically interpret results in a rapidly evolving methodological space.
More Posts from psychprofile.io: