AI-Powered Psychological Profiling - Gain Deep Insights into Personalities and Behaviors. (Get started for free)
Unveiling the Complexities of Human Language Processing Insights from the Academic Human Language Comprehension Test
Unveiling the Complexities of Human Language Processing Insights from the Academic Human Language Comprehension Test - Neural Architecture Complexities in Language Processing
Understanding how the brain processes language, extracting meaning from complex linguistic structures, remains a central challenge in cognitive neuroscience. While traditional methods like brain imaging and behavioral studies have provided valuable insights, a comprehensive mechanistic account of language comprehension has remained elusive. However, the field has seen a surge of interest in artificial neural networks, particularly advanced transformer models, as tools to investigate these neural processes.
These models, when combined with human brain activity data, are showing remarkable success in predicting and explaining neural responses to language. The ability of these models to account for nearly all the variance in neural activity during language tasks offers a powerful lens into the computations potentially occurring in the human brain. This convergence of AI and neuroscience is fostering a more integrated approach, where cognitive processes, brain activity, and behavioral outcomes are linked in a more coherent framework. Ultimately, this synergy may unlock a deeper comprehension of the intricate neural architecture that underlies human language comprehension.
Human language processing, a remarkably complex cognitive function, presents a compelling challenge for understanding how the brain translates sequences of words into meaningful representations. While traditional methods have provided insights, they often fall short of explaining the intricate mechanisms at play. Interestingly, the rise of artificial neural networks, particularly transformer architectures, offers a new lens through which to investigate these processes. These models, with their attention mechanisms, move beyond static representations of words, dynamically adjusting their focus based on the context in which words appear. This dynamic approach offers a fresh perspective on how meaning might be derived from language.
However, it's not simply about predicting the next word. These models appear to acquire an emergent grasp of syntax and semantics, allowing them to produce sentences that are both grammatically correct and contextually relevant. This emergent behavior suggests that deep neural networks may be capturing some fundamental aspects of language understanding, but the mechanisms are still not fully clear. The inherent ambiguity of language, where words and phrases can have multiple meanings, poses a major hurdle for machine learning algorithms. Unlike supervised tasks where labels clearly delineate the correct answer, language processing necessitates models to infer meaning without explicit instructions or context, a capability that remains challenging.
Furthermore, it's becoming evident that deeper networks aren't always the solution. We find that exceeding a certain complexity can lead to overfitting, highlighting a subtle relationship between model complexity and performance. This leads to questions about the optimal architectural design and the true impact of different layers. Researchers have found that incorporating multimodal data, such as visual information along with text, can bolster language processing. This observation mirrors human comprehension, suggesting we rely on more than just words to grasp meaning.
Current language models, however, still fall short in understanding pragmatics, the nuances of implied meaning, context, and speaker intention. Bridging this gap requires further exploration and, potentially, novel architectural designs. Clues from neuroscience offer potential directions. We know that specific brain regions like Broca's and Wernicke's areas are heavily involved in language processing, potentially providing guidance for better artificial models.
The rapid evolution of these architectures, though promising, has introduced ethical considerations regarding biases that can creep into models from their training data. This raises concerns about the potential for these models to unintentionally perpetuate and even amplify existing societal prejudices. There's also the intriguing question of how positional encoding contributes to the models' success. Researchers suggest that without incorporating the order of words, models might struggle with sequence-dependent tasks, which underscores the fundamental importance of sentence structure.
While unsupervised learning techniques have fueled significant advances in pre-trained language models, we are still facing challenges. The vast quantities of text data needed for training are a double-edged sword. While enabling impressive capabilities, this data dependency can limit a model's ability to generalize across diverse domains or speaker variations. This necessitates exploring more efficient training methods and architectures that can learn from a wider range of data sources and adapt to new contexts more readily. The journey towards truly understanding and replicating human language processing is a complex and exciting research frontier.
Unveiling the Complexities of Human Language Processing Insights from the Academic Human Language Comprehension Test - Challenges in Extracting Meaning from Language
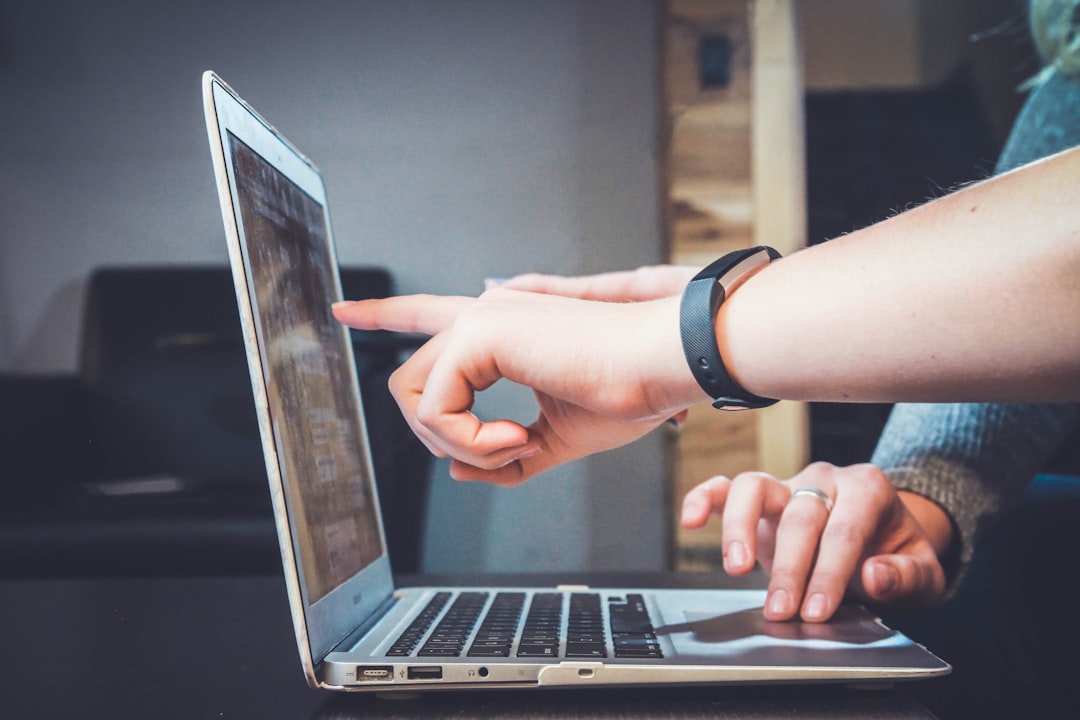
The process of extracting meaning from language presents a formidable challenge due to the intricate nature of human language itself. The way we produce and understand language are distinct yet interwoven processes, indicating that deriving meaning is a complex interplay between these two aspects. The inherent ambiguity of language, where words and phrases can have multiple interpretations, adds another layer of difficulty for both humans and machines trying to comprehend meaning. Furthermore, the context in which language is used heavily influences interpretation, adding a further layer of complexity. While progress in natural language processing has improved our ability to manage complex language, current models still grapple with understanding the subtle implications of meaning, including pragmatics and inferred context, highlighting the remaining gap in achieving truly comprehensive language comprehension. To bridge this gap and overcome the challenges of language understanding, researchers must not only develop advanced computational methods but also integrate insights from cognitive neuroscience to refine and improve artificial language processing systems.
Extracting meaning from language, while seemingly effortless for humans, presents significant hurdles for artificial systems. One key challenge lies in bridging the gap between a sentence's structure and its deeper meaning. Current models often struggle to effectively represent semantics, illustrating the intricate nature of translating linguistic patterns into conceptual understanding.
The inherent ambiguity of language, where words can have multiple meanings depending on context, further complicates matters. Unlike supervised learning scenarios with clear right and wrong answers, language processing requires models to infer meaning without explicit instructions. This ambiguity is a substantial barrier for current algorithms.
Interestingly, deeper isn't always better. We see that exceeding a certain complexity in neural network architectures can lead to decreased performance or overfitting, highlighting the importance of finding an optimal model design. This points to a subtle, and not fully understood, relationship between network complexity and its ability to generalize.
Human language processing often draws upon multimodal information, combining visual and textual input. However, current models are less adept at this integration. This limitation suggests that a truly comprehensive understanding of language, like our own, may involve synthesizing information across different modalities.
Furthermore, understanding the nuanced aspects of language like tone, idiom, and implied meaning (pragmatics) remains elusive. Current models struggle to capture these essential elements of human communication, underscoring the difficulty of replicating human-like subtleties in an algorithmic system.
Neuroscience provides clues about how humans process language, showing that different brain areas are active during various aspects of comprehension. However, mimicking these distinct patterns in artificial models remains a challenge. Understanding these neural interactions could be crucial for developing more effective language models.
The success of transformer models in handling language is closely linked to their incorporation of positional encoding. This highlights the crucial role of sentence structure in meaning extraction, mirroring our own cognitive reliance on word order.
Training language models requires enormous datasets, which, while enabling impressive capabilities, can limit a model's ability to adapt to new contexts and diverse linguistic variations. This dependence on vast datasets necessitates exploring more flexible training approaches that can learn from a wider variety of data.
Though language models exhibit emergent properties like the ability to generate grammatically correct and contextually relevant sentences, the mechanisms behind this learning are still not fully clear. This lack of clarity raises important questions about how these systems truly understand language compared to humans.
Finally, we must acknowledge the ethical considerations stemming from the potential for biases embedded within training datasets to influence model outputs. This risk underscores the need to address fairness and transparency in building language models, ensuring that they don't inadvertently perpetuate societal biases. The quest to replicate and fully understand human language processing is an exciting and complex journey, fraught with challenges and opportunities for innovative research.
Unveiling the Complexities of Human Language Processing Insights from the Academic Human Language Comprehension Test - Statistical Analysis of Written Language Structure
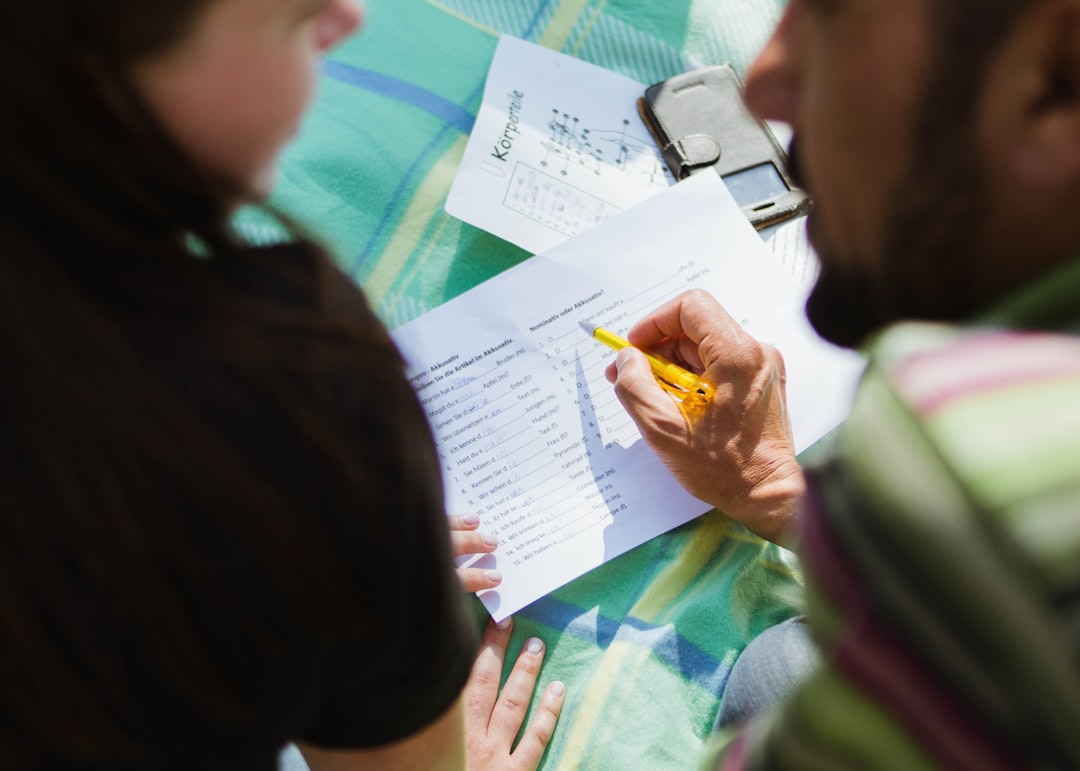
Examining the statistical properties of written language reveals a fascinating interplay between language structure, human cognition, and cultural evolution. Quantitative studies are increasingly demonstrating that the statistical patterns found within language aren't simply a consequence of its inherent complexity. Instead, these patterns are demonstrably influenced by cultural development, highlighting the deep connection between language and human societies.
These studies have also unearthed strong statistical trends, known as language universals, which are essential for developing explanatory theories of language structure. However, understanding these trends effectively demands innovative methods and approaches. Furthermore, the concept of language efficiency has emerged as a critical factor shaping communication, emphasizing the cognitive mechanisms at play in both understanding and constructing meaning.
Despite substantial progress, a complete and neurally grounded explanation of how we extract meaning from language remains elusive. This gap represents a major challenge at the intersection of computational models and neuroscience. As researchers continue to explore the statistical aspects of language, they encounter significant methodological and theoretical challenges that have the potential to reshape our understanding of how humans process language.
Statistical analysis of written language is revealing unexpected patterns that challenge our conventional understanding of language structure and complexity in human communication. Language structure appears deeply intertwined with cultural evolution, suggesting a fascinating interplay between linguistic development and human societies. Human language possesses a unique property: its components can be recombined in countless ways, leading to a vast expressive potential. This combinatorial nature presents a significant challenge to language learners, both human and artificial, as they must discover the underlying structure to effectively process and produce language.
Interestingly, across languages, we observe strong statistical tendencies – language universals – that are proving useful in developing theoretical frameworks to explain the fundamental principles governing language structure. Efficiency seems to play a key role in shaping how we communicate, implying that efficient communication is a driving force behind both linguistic and cognitive processes.
Despite significant research using brain imaging and computational modeling, understanding how the brain extracts meaning from language at a neural level remains elusive. We can quantify comprehension difficulty by analyzing metrics like reading times and event-related potential (ERP) magnitudes, which offer a window into the cognitive processes involved in language understanding.
One recent large-scale effort involved training a language model on a massive dataset of over 6,500 documents from 41 multilingual collections, representing approximately 35 billion words. This cross-linguistic analysis is providing insights into the statistical similarities and differences across languages.
Examining language complexity presents both methodological challenges and profound implications for linguistic theories. A recent surge of research has focused on language efficiency, exploring its role in shaping language processing and structure. This exploration is leading to new questions about how optimization for efficiency might have impacted the evolution of language and its underlying neural architecture.
However, many open questions remain regarding the optimal design for language models. The search for truly effective language comprehension models is an ongoing effort, and as new methods and data are explored, we're gaining a deeper understanding of the complexities of human language and the computational challenges involved in building artificial systems that can replicate this remarkable ability.
Unveiling the Complexities of Human Language Processing Insights from the Academic Human Language Comprehension Test - Machine Learning Applications in Sentence Activation
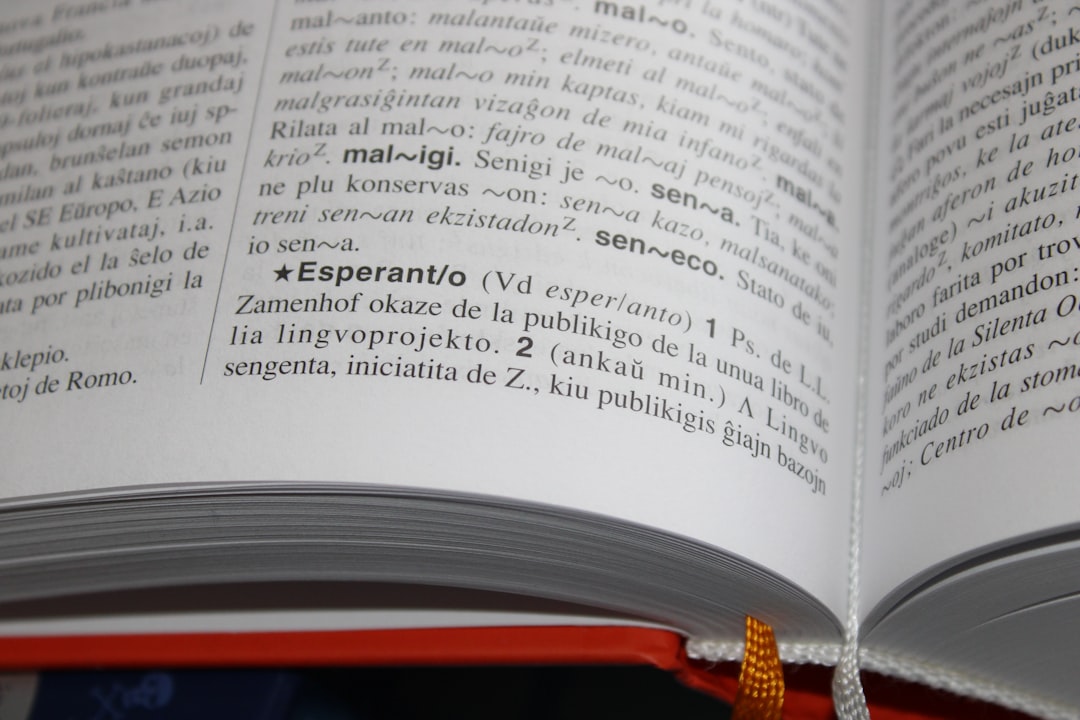
Machine learning is finding increasing utility in the study of sentence activation, a crucial aspect of human language processing. By employing sophisticated neural network architectures, researchers are investigating how these models can identify and manipulate sentence structures to either amplify or diminish activation within the brain's language processing networks. While these computational methods hold promise in reflecting certain facets of human cognition, substantial gaps remain, especially in capturing the intricate and nuanced aspects of meaning and context inherent in human language. As these models become more complex, a careful evaluation of their ability to generalize and effectively handle the subtle complexities of pragmatic language use becomes critical. This fusion of machine learning and cognitive neuroscience presents both exciting possibilities and formidable challenges in the comprehensive exploration of the intricate workings of human language processing.
Machine learning, particularly deep learning approaches, has opened up new avenues for studying how sentences are activated within the human language processing network. We can now leverage these computational tools to try and understand the mechanisms of sentence comprehension.
One fascinating development is how these newer transformer-based language models handle sentence context. Instead of relying on static representations of words, these models dynamically adjust their understanding based on the words and phrases surrounding a sentence. This dynamic contextualization aligns with how we as humans seem to process language in real-time, constantly adjusting our interpretations based on what's happening around the sentence.
Transformer models also employ attention mechanisms which seem to parallel how humans prioritize certain aspects of a sentence when deriving meaning. These models can essentially focus on the most important parts of a sentence – helping them deal with ambiguity and complex sentence structures that would otherwise be problematic.
However, there are also surprising challenges. For example, these models, even with extensive training on huge datasets, sometimes fail to apply their knowledge to new and unfamiliar situations. This generalization challenge suggests that having more training data doesn't always guarantee better performance, and the relationship between the amount of data and generalizability remains an open question.
Another intriguing development is that activation patterns observed in these deep learning models bear some resemblance to brain activity patterns found in humans during language tasks. This raises the possibility that these models may indeed be capturing aspects of how humans process language at a cognitive level, albeit in a simplified fashion.
Through analysis of massive text datasets using machine learning, we've been able to identify patterns called language universals, which are characteristics found across various languages. This is helping us better understand the building blocks and potential organizing principles of language from a cognitive and structural perspective.
Looking at efficiency in how these models process sentences, we find that shorter, more concise sentences are often easier and faster for the models to process. This aligns with human language tendencies and strengthens the idea that computational models could help us understand language evolution, from both a cognitive and a social/communication perspective.
Additionally, research shows that adding other modalities, such as visual input, can benefit language models, a finding that echoes human language comprehension where context and sensory experiences often play a crucial role. This highlights the potential for future AI designs that incorporate a more comprehensive understanding of language in context.
A troubling discovery has been the unintended consequences of training models on vast datasets – the risk of absorbing and reflecting any bias embedded within that data. This can affect how sentences are activated and interpreted, which necessitates careful attention to the datasets used and ethical considerations related to fair and unbiased AI development.
We also see that positional encoding is absolutely necessary for models to understand language. If you don't tell the model the order of the words in a sentence, the model struggles to understand what it means. This highlights a fundamental aspect of sentence structure in both human and machine language processing.
In some cases, language models exhibit something called emergent syntax. They not only generate grammatically correct sentences, but also contextually appropriate ones, revealing that these models might be grasping fundamental linguistic principles that are still not fully understood. This emergent behaviour raises new questions about how machines might learn language and how their understanding might differ from ours.
In summary, machine learning and particularly the recent developments in deep learning offer a promising range of new tools for exploring sentence activation in human language comprehension. However, the relationship between models and the brain remains under investigation, and there are many challenges and ethical considerations related to this field of study.
Unveiling the Complexities of Human Language Processing Insights from the Academic Human Language Comprehension Test - Multilayered Framework for Rapid Language Processing
The concept of a "Multilayered Framework for Rapid Language Processing" suggests a way to understand how deep language models might mirror the human brain's rapid language processing abilities. This framework highlights the need for models that can handle various types of information – like text, pictures, sounds, and even biological signals – to tackle complex tasks in the real world. Interestingly, studies show that humans can process information faster when it comes from multiple sources (like seeing and hearing someone speak), compared to just one source. This idea presents both a potential advantage and a major challenge for AI. If AI systems are to truly understand language like we do, they need to quickly combine different types of information. This framework encourages researchers to look closely at both the general and specific cognitive processes humans use to understand language. The link between AI and the study of the human brain offers a fascinating opportunity to improve our understanding of the intricacies of how we process language. While the current generation of AI models shows promise, further research is crucial to replicate and improve these intricate cognitive abilities.
1. **Navigating the Layers**: In complex, multilayered neural networks, how layers interact significantly affects how well they perform. While we might expect deeper networks to handle more intricate linguistic features, there's a surprising possibility of overfitting, where increasing the number of layers actually harms the model's ability to generalize. This creates an intriguing dilemma around the ideal architecture for optimal results.
2. **Echoes of the Human Brain**: It's remarkable that the activation patterns within transformer models show similarities to brain activity observed in humans while processing language. This suggests these models might not just be imitating language superficially but might be capturing some of the core cognitive mechanisms that humans use.
3. **A Dynamic Understanding of Context**: Unlike older models that treated word meanings as static, multilayered frameworks adapt to the context dynamically. This more closely mimics how humans constantly adjust their interpretations based on surrounding words, showing a level of nuance missing in older NLP approaches.
4. **The Mystery of Emergent Syntax**: We see multilayered models showing emergent properties—they appear to grasp rules of syntax, generating grammatically correct and contextually relevant sentences. However, the underlying mechanisms behind this understanding remain obscure, leaving us questioning the extent to which they truly comprehend language and how this 'understanding' develops.
5. **The Importance of Word Order**: Positional encoding, which provides information about the order of words in a sentence, significantly boosts the performance of these models. This highlights that understanding the sequence of words isn't simply an added feature—it's a crucial part of effective language processing for both machines and humans.
6. **Data's Limits on Generalization**: Interestingly, having more training data doesn't always result in improved generalisation in multilayered models. This raises fundamental questions about the quality and diversity of training data, indicating that the model's architecture might play a key role in determining what it learns.
7. **The Power of Multimodal Input**: Adding visual context to textual data seems to improve activation within neural models, which mirrors how humans often rely on multisensory cues when interpreting language. This highlights a potential path for future AI systems—integrating multisensory information for a more comprehensive understanding of language.
8. **Measuring Cognitive Load**: Researchers are using measures like reading times and neural activations to study comprehension difficulty in both models and humans. This comparative approach opens up possibilities for examining how layers in a network might map to or distort the cognitive load involved in language processing.
9. **Language's Connection to Culture**: Statistical analysis within these complex frameworks has revealed strong links between the intricacy of language and the evolution of cultures. Understanding these correlations could significantly impact our understanding of language from the perspectives of both linguistics and cognitive science.
10. **Addressing Bias in Language Models**: The fact that biases present in training data can influence the output of these models underscores ethical concerns. These biases can subtly affect how sentences are interpreted, creating a need for careful attention to data quality and the development of techniques to mitigate biased outcomes in machine learning.
Unveiling the Complexities of Human Language Processing Insights from the Academic Human Language Comprehension Test - Integrating Words and Sentences for True Comprehension
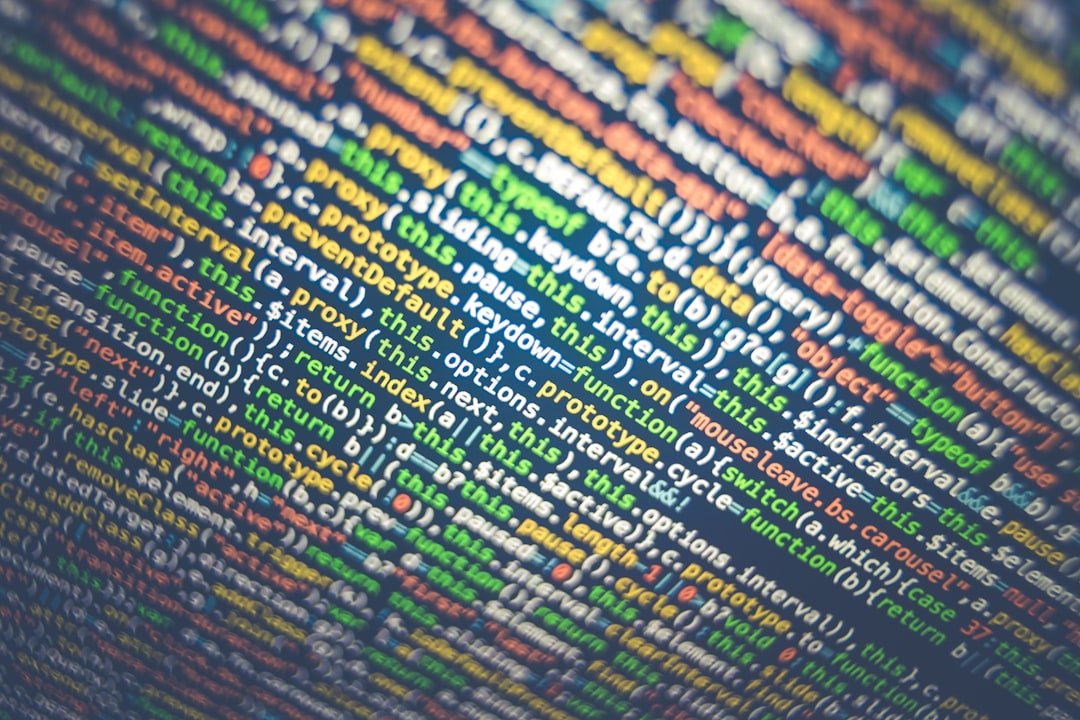
Understanding how we integrate words and sentences to grasp meaning is a complex cognitive feat. It involves not only deciphering individual word definitions but also recognizing how these words relate to one another within sentences and larger contexts. The context surrounding language is crucial, shaping both our grammatical understanding and the meanings we assign to words as we build coherent mental representations of the information we encounter. While progress in artificial language processing has brought us closer to understanding this process, there are still significant challenges. Specifically, replicating the nuanced aspects of human comprehension, such as understanding implied meanings and the subtle nuances of context, remains a significant obstacle. Bridging this gap is fundamental for developing more sophisticated language models that can effectively mimic human-like comprehension in artificial intelligence systems. The journey towards replicating this sophisticated ability is an ongoing challenge that requires ongoing research and development.
1. **Complexity's Trade-offs**: It's fascinating that deeper neural networks aren't always the answer in language processing. While we might think more layers would lead to better understanding, it can lead to the model fitting the training data too closely, and thus not being able to handle new or unseen language ("overfitting"). This highlights the importance of carefully considering model design, not just adding more layers for the sake of it.
2. **Mirroring Brain Activity**: It's quite intriguing that the activation patterns we see in advanced language models seem to echo what happens in the human brain during language tasks. This raises the question of whether these models are just mimicking language superficially, or if they might actually be capturing some of the fundamental cognitive processes we use to understand language.
3. **Contextual Understanding**: Older language models treated words like they always had the same meaning, but newer models can change their interpretation based on the surrounding words and the context of the sentence. This dynamic adjustment is much closer to how humans process language, constantly refining our understanding based on the current situation. It's an important step forward in making language models more like how we think.
4. **The Mystery of Emergent Grammar**: Some complex language models seem to develop their own "grammar" – they can generate sentences that are both grammatically correct and relevant to the situation. This ability is pretty surprising, but we don't entirely understand how it happens. It raises questions about how these models truly "learn" language and if their understanding is the same as ours.
5. **The Order Matters**: The way words are ordered in a sentence is crucial for meaning, and these models show this clearly. If you don't give the model information about the order of words, it has trouble understanding what the sentence means. This reinforces how sentence structure is fundamental for both humans and machines when interpreting language.
6. **Data Quality is Key**: It's not just about having more training data for language models; the diversity and quality of the data also matter. More data isn't always better, and it seems like the model's design also plays a big role in how well it generalizes to new situations. This emphasizes that effective language model learning isn't solely dependent on quantity but also on the nature of the training data.
7. **Seeing and Hearing for Better Understanding**: Just like us, language models seem to benefit from incorporating multiple sources of information. For example, combining images with text can greatly improve a model's performance. This observation mirrors our reliance on sensory cues in understanding language and shows a promising path for future AI that can interpret language more comprehensively.
8. **Measuring How Hard it is to Understand**: We can use things like reading times and brain activity measurements to see how hard it is for both humans and AI to understand language. By comparing these, researchers can begin to understand how different parts of a language model relate to the effort we put into understanding language. It's a vital area for future research.
9. **Language and Culture**: Statistical studies show that the complexity of a language seems to be connected to the way a culture evolves. This connection is quite interesting and could lead to a better understanding of both language and the dynamics of cultural development. It could contribute significantly to the fields of linguistics and cognitive science.
10. **Bias Can Creep In**: A worrisome aspect of these language models is that they can pick up on and reflect biases present in the data they're trained on. This can subtly affect how the models interpret language and raises serious ethical questions about how we develop and use AI in language processing. It's important to be mindful of this issue and work towards developing unbiased AI systems.
AI-Powered Psychological Profiling - Gain Deep Insights into Personalities and Behaviors. (Get started for free)
More Posts from psychprofile.io: